Build or Buy AI? A Guide
The AI Dilemma
In today’s business world, artificial intelligence (AI) is no longer science fiction but a key to innovation, efficiency, and competitive advantage. From automating mundane tasks to data-driven decision-making, the potential of AI is huge. But as organizations look to leverage AI they have a big decision to make: should they build AI in-house or buy off-the-shelf AI from vendors?
This article goes into the details of this decision, the factors you need to consider, the pros and cons of each approach, and the possibility of a hybrid approach.
1. Business Context
Before we get into the build vs buy debate, you need to understand your business context. AI is not a one-size-fits-all solution; the right approach depends on several key factors:
1.1. Business Goals and Strategic Alignment
The first step in any AI strategy is to align it with your overall business objectives. What problems do you want AI to solve? Are you looking to improve customer experience, optimize operations, or create new revenue streams? Clearly define your goals and that will help you determine if you need a custom in-house solution or if an existing product will do the job.
1.2. Industry and Competition
Think about the specific challenges in your industry and how competitors are using AI. In highly competitive spaces having a unique AI solution can be a big differentiator. In industries where AI is still in its infancy an off-the-shelf solution may be enough.
1.3. Organizational Readiness
Assess your organization’s readiness for AI. This includes the maturity of your data infrastructure, the availability of skilled people, and the openness of your culture to change. The decision to build or buy should be influenced by your current capabilities and the resources you are willing to invest in AI development.
2. The Economics of AI: Cost
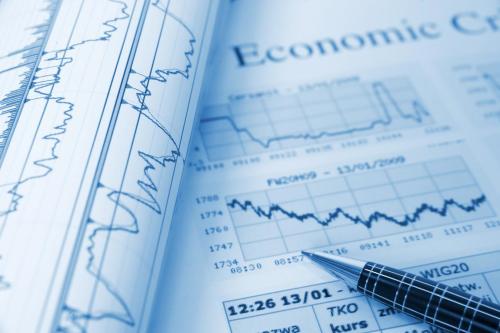
Cost is often the biggest factor in the build vs buy decision. However, the financial implications go beyond the initial cost.
2.1. Upfront
Building an AI solution in-house requires a big upfront investment. This means hiring or upskilling a team of data scientists, engineers, and other specialists and investing in the necessary hardware and software infrastructure. Buying an AI solution often means a lower upfront cost but this can vary depending on the complexity and scope of the solution.
2.2. Ongoing Maintenance and Support
The cost of maintaining and updating an AI system can be high especially if you build it in-house. You’ll need to monitor the system, update models, and ensure compliance with changing regulations. Purchased solutions often include support and updates as part of the vendor agreement but this may come with recurring fees.
2.3. Hidden Costs
Both build and buy have hidden costs. For in-house solutions, these might be development delays, additional hires, or the cost of scaling the solution. For purchased solutions, the hidden costs might be integration with existing systems, customization fees, or the cost of switching vendors if the solution doesn’t meet expectations.
3. Time to Market: Speed vs Customization
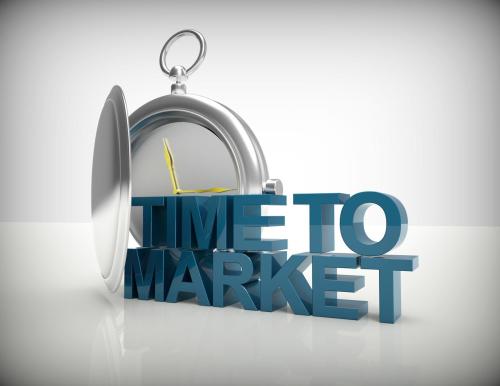
In today’s fast-paced business environment, time to market is a key consideration.
3.1. Build: The Long Road
Building a custom AI solution is a time-consuming process. It involves not only developing the technology but also training it on your data, testing it, and refining it based on real-world performance. This gives you a solution tailored to your needs but requires patience and a long-term commitment.
3.2. Buy: Fast Deployment
Buying an AI solution means faster deployment. Many vendors offer pre-trained models that can be plugged in quickly. This is especially useful if you need to address an immediate business need or if time to market is a competitive factor. But you may have to compromise on flexibility and customization compared to a build solution.
4. Talent and Expertise: The Human Element
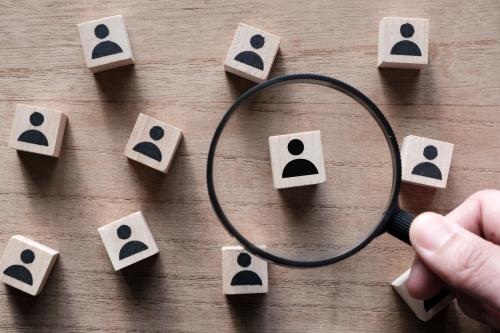
AI is as much about people as it is about technology. The decision to build or buy is about the talent within your organization.
4.1. Building Requires a Specialized Team
Building an AI solution from scratch requires a highly specialist team. This includes data scientists, machine learning engineers, software developers and domain experts who can translate business needs into technical requirements. Attracting and retaining this talent can be tough, especially in a competitive job market. Managing an AI team also requires leadership that understands both the technical and strategic aspects of AI.
4.2. Buying Leverages External Expertise
Buying an AI solution means you’re relying on the expertise of external vendors. This is useful if your organization doesn’t have the in-house talent to develop AI. Vendors often provide end-to-end solutions from deployment to ongoing support so your team can focus on other strategic areas. However, this approach can lead to dependency on external providers and limit your ability to innovate independently.
5. Scalability and Flexibility: Future Proofing
AI solutions need to be scalable to grow with your business and flexible to adapt to changing needs.
5.1. Scalability of In-House Solutions
Building an AI solution in-house means you can design for scalability from the start. You can create an architecture that grows with your data, users, and computational requirements. However, designing for scalability requires a lot of foresight and technical expertise. Any mistakes in the design phase will cost you dearly down the line.
5.2. Flexibility of Bought Solutions
Purchased AI solutions offer varying degrees of scalability and flexibility. Many vendors provide cloud-based solutions that scale effortlessly as your business grows. However, these solutions may not offer the same level of customization as a build solution. If your business needs change dramatically you may find yourself constrained by the limitations of a bought solution.
6. Intellectual Property and Competitive Advantage
Intellectual property (IP) is a big consideration if AI is core to your competitive strategy.
6.1. IP in Building
When you build an AI solution in-house you retain full ownership of the intellectual property. This is a big advantage if the AI provides a unique value proposition that sets you apart from competitors. Owning the IP means you have control over how the technology is used, modified, and commercialized in the future.
6.2. IP in Buying
When you buy an AI solution the IP usually stays with the vendor. This means you’ll be using the same technology as your competitors and potentially losing your competitive advantage. However, some vendors offer co-development agreements or licensing arrangements that give you more flexibility over IP rights.
7. Hybrid Approach: The Best of Both Worlds?
For many organizations the best approach will be a hybrid approach, combining elements of both building and buying AI.
7.1. Build the Core, Buy the Rest
One hybrid approach is to build the core AI capabilities that are core to your business and buy the rest. For example, you might build a proprietary machine-learning algorithm in-house and buy data management tools from a vendor.
7.2. Hybrid Case Studies
Many organizations have implemented hybrid approaches. For example, a financial services firm might build custom algorithms for trading and buy an AI platform for customer service automation. This allows them to maintain a competitive edge in their core area and benefit from pre-built solutions in other areas.
8. Decision-Making Framework: A Strategic Approach
Making the right decision requires a structured approach. Here’s a framework to help you decide:
8.1. Organization Readiness
Infrastructure: Do you have the data infrastructure?
Talent: Do you have the necessary skills?
Culture: Is your organization open to new technology?
8.2. Strategic Importance
Core Competency: Is AI core to your competitive advantage?
Long-Term Vision: How will AI fit into your long-term strategy?
8.3. Costs and Benefits
Total Cost of Ownership: Consider both upfront and ongoing costs.
Risk Assessment: What are the risks of each approach and how can they be mitigated?
8.4. Pilot Programs
Test and Learn: Consider running a pilot to test both build and buy. Use this as an opportunity to gather data and insights to inform your decision.
Conclusion: Make It Your Own
The build or buy decision is not a straightforward one. It requires consideration of your organization’s objectives, resources, and the broader competitive landscape. Building gives you a bespoke solution that aligns with your business needs but comes with higher costs and risks. Buying gives you speed and access to expertise but limits customization and scalability.
In the end, it’s up to you. Follow the framework above and consider a hybrid approach and you’ll make a strategic decision for your organization to thrive in the AI world.