Generative AI: A Brief History
The history of generative AI traces a fascinating journey from its inception in the mid-20th century to the sophisticated models we see today. This article dives into the key milestones and breakthroughs that have defined generative AI, offering insights into its origins and major developments.
Key Takeaways
Mid 20th century: Generative AI was born with early neural network concepts and baseline models like Hidden Markov Models and Gaussian Mixture Models.
1960s and 1970s: ELIZA and pattern recognition laid the foundation for modern generative AI.
Modern generative AI (GANs and ChatGPT) has enabled text, image and audio generation and we’re just getting to the ethics and regulation part.
Generative AI’s Origins
Generative AI has a fascinating history that starts in the mid 20th century. It was the time when AI was born and machine learning algorithms were sprouting. Tech pioneers wanted to build machines that could not only learn but also generate new, unseen work. This led to the creation of generative models like Hidden Markov Models and Gaussian Mixture Models and the use of neural networks which became the foundation of generative AI.
These early developments led to the generative AI models that today are woven into the fabric of modern technology with the help of a generative AI tool.
Early Machine Learning Algorithms
The concept of neural networks was proposed by Warren McCullough and Walter Pitts in 1944. It was revolutionary, but the first neural network had many limitations due to the computational power and data availability of the time. But these early neural networks were the precursor to the sophisticated generative AI tools that would later change the game of AI.
Minsky and Papert’s ‘Perceptrons’ in the late 1960s raised criticisms of single layer neural networks and cast a shadow of doubt over the field. But machine learning algorithms were resilient and adaptable and this was just a bump in the road for generative AI.
Artificial Intelligence is Born
The Dartmouth Summer Research Project on Artificial Intelligence in 1956 is where the term ‘artificial intelligence’ was coined and the field was born. This gathering of geniuses kicked off a movement that would extend human thinking into the machines and lead to the development of generative ai models that could mimic human intelligence and creativity.
This was more than just a naming of a new field, it was the ambition to combine human expertise with machine power. It led to the creation of generative ai tools that could learn from all the human knowledge and generate ai generated art, content and solutions that are inspired by but not limited by human imagination.
1960s and 1970s
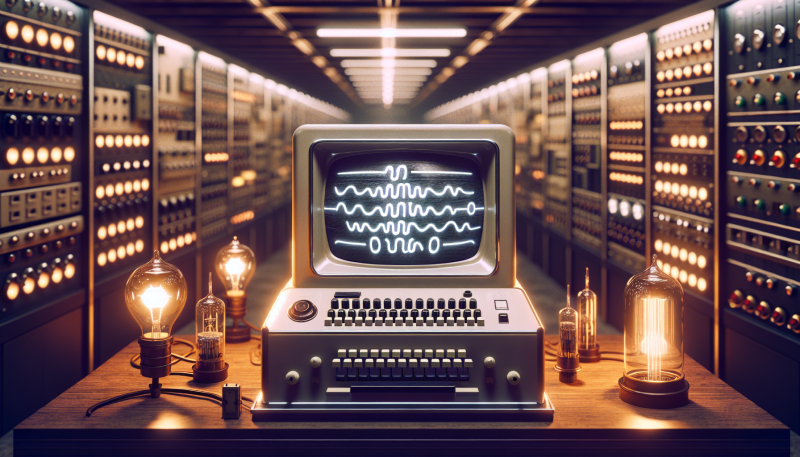
The 1960s and 1970s saw the pioneering developments in generative AI. ELIZA, the talking computer program was born and pattern recognition made big strides.
These early developments set the stage for the generative models including large language models that would later change industries and the way we interact with technology.
ELIZA: The First Talking Computer Program
ELIZA was developed by Joseph Weizenbaum at MIT in the 1960s and was the first program to mimic human conversation through natural language processing. It could engage users in simple dialogue and create the illusion of understanding human speech. ELIZA was a simulated psychotherapist and was not only a technical achievement but also a social experiment to show how much users could bond with a machine.
ELIZA’s genius was in its simplicity and the implications for conversational AI. It showed that language models and virtual assistants could one day understand and respond to human speech in ways previously thought impossible.
Pattern Recognition
The 1960s and 70s saw huge advances in facial recognition. Researchers like Ann B. Lesk, Leon D. Harmon and A. J. Goldstein improved the technology by using specific markers to increase recognition accuracy. This was:
fertile ground for innovation
big advances
Ann B. Lesk, Leon D. Harmon, A. J. Goldstein
the computer vision systems we have today
Also Seppo Linnainmaa’s backpropagation technique introduced in the 1970s was a major breakthrough for training neural networks. By moving the errors backwards through the layers it was possible to improve the model’s accuracy and speed. These early pattern recognition developments paved the way for the modern generative ai models that can create realistic images and process huge amounts of data with unprecedented precision.
The AI Winters and Their Consequences
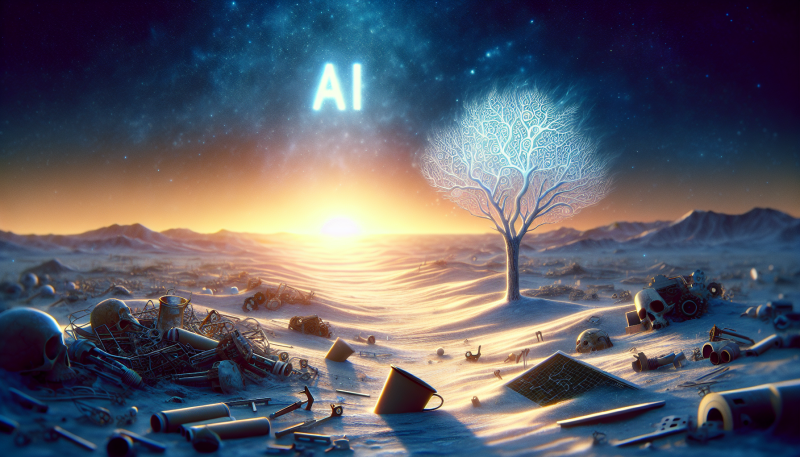
The journey of AI has not been smooth. The field has had its ups and downs, known as AI winters, where the enthusiasm and investment in AI research has waned due to unmet expectations and the complexity of the goals.
These winters were short but had a big impact on the funding and progress of generative AI.
The First AI Winter
The first AI winter was between 1974 and 1980. It was triggered by the Lighthill report which was pessimistic about the progress of AI. The report and the publication of ‘Perceptrons’ led to a big cut in funding as DARPA and other agencies stopped supporting AI research. The effects were felt across the board as the British government and the National Research Council also reduced their support for AI and put the future of AI into question.
This was a period of disappointment and skepticism as the initial hype about AI faded and a more realistic approach took over. The first AI winter was a wake up call about the complexity of human intelligence and the need for a more modest expectation of what AI can do.
The Second AI Winter
The second AI winter was between late 1980s to mid 1990s and was marked by:
Further funding cuts
Big decline in interest
The Strategic Computing Initiative which had poured resources into AI projects earlier scaled back their support drastically
The collapse of the LISP machine market in 1987 and the decline of commercial interest in expert systems by early 1990s made the situation worse and led to a big reduction in AI research funding
The Japanese Fifth Generation project which was ambitious but failed also contributed to this downturn.
But this tough period was followed by a resurgence in AI research with the introduction of backpropagation and as the second AI winter thawed it was clear that the cycles of hype and disappointment was part of the maturing process and the foundation for future growth.
Resurgence and Growth in 1990s
The 1990s was a turning point for AI as the field experienced a resurgence with a combination of factors including more computing power and new methodologies. Support vector machines and recurrent neural networks emerged and that paved the way for a new era of AI research and applications.
Boosting
One of the key methodology of 1990s was the concept of ‘boosting’ introduced by Robert Schapire. Boosting techniques like AdaBoost developed in 1996 combined the strengths of multiple weak learners into a strong classifier. AdaBoost was a big deal as it showed that an ensemble of simple models can outperform a single complex model.
Boosting techniques embodied the collaborative spirit of AI research, that collective intelligence even among algorithms can lead to better performance and efficiency. This approach to machine learning would be the foundation for future generative AI tools.
Contributions from Gaming Industry
The 1990s also saw the gaming industry make an unexpected but important contribution to AI. The development of 3D graphics cards for gaming purposes led to a big increase in computing power for AI research. The symbiotic relationship between gaming and AI was a proof that innovation in one industry can benefit another.
The hardware from 3D graphics cards not only boosted AI capabilities but also lowered the barrier to entry for researchers and developers. The increased computing power enabled more complex and nuanced generative AI models which would later be used for image generation and modern generative AI.
Breakthroughs in Early 2000s
Technological advancements in early 2000s with the rise of Internet and increase in computing power enabled new breakthroughs in AI. Among these was the Face Recognition Grand Challenge which pushed the limits of facial recognition and the rise of deep learning which would redefine the capabilities of AI systems.
Face Recognition Grand Challenge
The Face Recognition Grand Challenge was held from May 2004 to March 2006. It was an effort to significantly improve face recognition systems. It provided researchers with large datasets and challenging problems to solve and overcome previous hurdles. FRGC was instrumental in improving facial recognition systems and introduced techniques to recognize identical twins.
The FRGC results were significant, high resolution images, 3D recognition, new preprocessing techniques to handle lighting and pose changes. These would not only advance computer vision but also the foundation for generative AI tools to build upon for image generation and beyond.
Rise of Deep Learning
Deep learning, a subset of machine learning, grew rapidly in early 2000s. Neocognitron proposed by Kunihiko Fukushima in 1979 was the precursor to the deep learning neural networks that would later become the backbone of AI. Backpropagation, essential for training these networks was refined to improve their learning and processing capabilities.
Recurrent Neural Networks (RNNs) and its variants like Long Short-Term Memory (LSTM) networks were key for sequential data tasks like speech recognition and machine translation. These deep learning architectures enabled AI systems to process and generate content with depth and complexity of human intelligence, pushing the limits of artificial neural networks.
Modern Generative AI (2010s - Present)
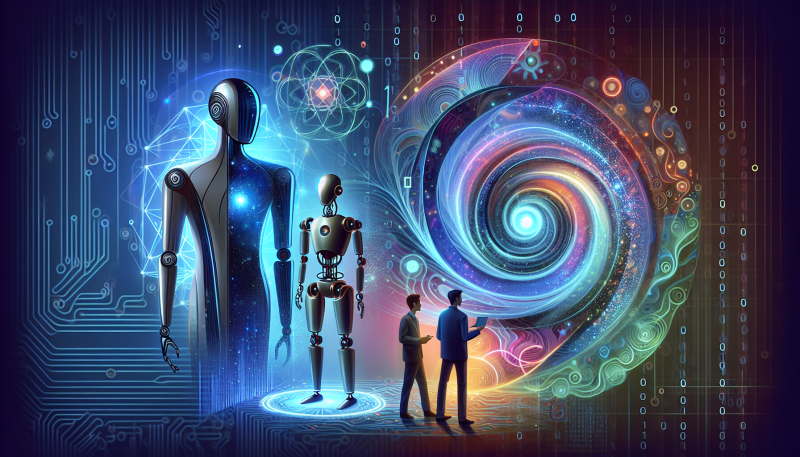
2010s was the modern era of generative AI, with breakthroughs like virtual assistants, Generative Adversarial Networks (GANs) and introduction of transformative technologies like OpenAI’s ChatGPT. This decade has seen unprecedented growth in generative AI capabilities and applications, with models that can now generate text, images and even audio that are indistinguishable from human created content.
Virtual Assistants and Chatbots
Virtual assistants like Siri introduced in 2011 changed the way we interact with our devices by using generative AI models to have natural conversations and answer questions. These assistants use advanced machine learning algorithms to process natural language text and respond to prompts to provide seamless human-computer interaction.
Virtual assistants and chatbots are everywhere in our daily lives, providing assistance, entertainment and even companionship. It’s a testament to the progress in natural language processing and generative AI models.
Generative Adversarial Networks (GANs)
Generative Adversarial Networks (GANs) introduced in 2014 by Ian Goodfellow is a major milestone in AI’s ability to generate synthetic data. GANs work by pitting two neural networks against each other. One network generates content, the other evaluates its authenticity. This competition makes the generative network to produce more and more realistic outputs:
Images
Audio
Text
Videos
The applications of GANs are endless, from generating realistic images for video games to creating deepfakes. GANs are also used in healthcare, finance and art.
GANs have evolved fast, from image generation to deepfakes. Their ability to generate high resolution and photorealistic content has opened up new possibilities in art, design and entertainment and raised important questions about authenticity and misuse.
OpenAI’s ChatGPT
OpenAI’s ChatGPT launched in 2022 is a milestone in conversational AI, where machines can have fluid and coherent conversations, answer complex questions and generate content across different domains. Its understanding of natural language and ability to generate natural language text makes it a versatile tool for tasks from customer support to creative writing. ChatGPT and its variants is a big part of modern generative AI, showcasing the power of generative pre-trained transformers to understand and generate human language at scale.
ChatGPT’s one million users in just 5 days of launch is a big moment for the public’s acceptance of advanced AI. It’s not just about text generation, it’s a demonstration of how AI can interact with users in a way that was once the exclusive domain of human intelligence. So ChatGPT has not only captured the world’s imagination but also set a new bar for what generative AI can do.
Generative AI Future
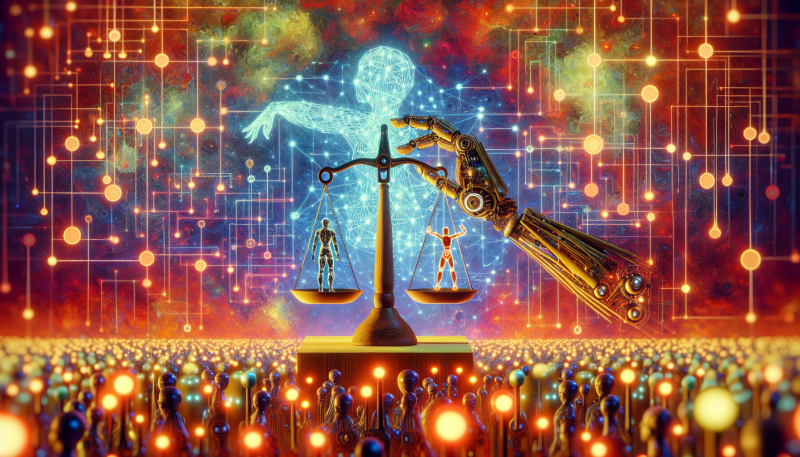
We are at the beginning of a new chapter of generative AI and the future promises to be transformative across many industries. Generative AI can disrupt the labor market, revolutionize content creation and change the way we interact with technology and redefine human-machine collaboration.
But this future also comes with ethical and regulatory challenges that we need to navigate carefully to make sure the benefits of generative AI is responsible and fair.
Disruptions
Generative AI is evolving fast, handling multiple input and output formats and changing the way we work by automating routine tasks and creating new opportunities for innovation. As businesses adopt AI-as-a-service models, they can get access to advanced AI without heavy infrastructure investment, even small businesses can join the AI bandwagon. Embedded AI in enterprise and customer facing tools will become more common, making user experience and workflows better. But with this transformation comes the responsibility to manage the ethics, job displacement and AI output accuracy.
AGI is a hotly debated and lofty goal in the AI community. There is no consensus on what it means or how to achieve it. But if we get there, we will have machines that are as intelligent as the human brain. As we move forward we need to stay informed and agile to the changes and opportunities brought by generative AI and make sure its disruption is for the good of the society.
Ethics and Regulations
Generative AI implementation raises big questions on data privacy, security and ethical use. As these tools get more into our lives, we need to develop robust strategies to protect sensitive information and ensure responsible use of AI. With the power of generative models increasing, we need a thoughtful approach that is built on trust and has safeguards against misuse.
Regulatory measures like the EU AI Act are emerging to address these concerns and govern the use of AI and data privacy. As generative AI continues to advance, it must do so within a framework that puts ethical considerations first and benefits all stakeholders. The future of generative AI should be shaped not just by technological progress but by societal values and the public good.
Conclusion
From neural networks to GPT-3 and beyond, the history of generative AI is a story of innovation, setbacks and resurgence. As we have gone through the key milestones and developments, it’s clear that generative AI has not only expanded the boundaries of what machines can do but also raised new questions on human-machine collaboration. We need to balance the potential of generative AI and its complexities but the possibilities are endless.
FAQs
What was the Dartmouth Summer Research Project on Artificial Intelligence in 1956 about?
The Dartmouth Summer Research Project on Artificial Intelligence in 1956 was the birth of AI as a named field of study and the precursor to the generative AI models and tools we see today. It defined the field of AI.
How did ELIZA impact conversational AI?
ELIZA contributed to conversational AI by being the first computer program to mimic human conversation through natural language processing. It laid the foundation for advanced language models and virtual assistants.
What are GANs and why are they important?
Generative Adversarial Networks (GANs) are machine learning models that use two competing neural networks to generate content. They are important because they have accelerated the progress of AI by allowing creation of synthetic data that is often indistinguishable from real data.
What impact did the AI winters have on generative AI?
The AI winters slowed down generative AI development due to lack of interest and funding but also made us more realistic and ultimately contributed to more progress in AI.
What are the ethical and regulatory issues with generative AI?
Generative AI has data privacy, security, job displacement and responsible use concerns. Need to regulate carefully to benefit society. Need to think and regulate.