Unveiling Future: Generative Artificial Intelligence & Evolution of AI
Introduction
In the rapidly advancing landscape of artificial intelligence, a new paradigm is emerging that promises to redefine our understanding of intelligence itself. Welcome to the era of Generative AI, where the boundaries between human and machine cognition blur, and the trajectory of technological progress takes on a profoundly human dimension.
Gen AI, shorthand for Generative Artificial Intelligence, represents a transformative leap forward in AI capabilities. Unlike its predecessors, which were confined to executing pre-defined tasks within narrowly delineated domains, Generative AI possesses the capacity for creativity, adaptability, and autonomous machine learning models. It stands poised at the threshold of a new era, where machines not only mimic human intelligence but also augment and extend it in ways previously unimaginable.
At its core, Generative AI embodies the synthesis of cutting-edge technologies such as deep learning, reinforcement learning, and generative adversarial networks (GANs). These technologies converge to imbue AI systems with the ability to perceive, reason, and create in a manner that mirrors human cognitive processes. Yet, what sets Generative AI apart is its capacity for self-improvement and co-creation with human counterparts, leading to a symbiotic relationship between man and machine.
In this article, we delve into the multifaceted landscape of Generative AI, exploring its origins, capabilities, and implications for society at large. From its roots in academic research to its burgeoning applications across industries, we uncover the pivotal role that Generative AI plays in shaping the future of technology and humanity's relationship with it. Moreover, we confront the ethical, societal, and existential questions that arise as AI transcends its role as a mere tool and evolves into a true partner in the human quest for progress.
Join us on a journey into the heart of Generative AI, where innovation knows no bounds, and the boundaries between science fiction and reality blur evermore. Brace yourself for a glimpse into a future where the distinction between human and artificial intelligence becomes increasingly indistinct, and the possibilities for collaboration and co-creation are limitless.
How does generative AI work?
Generative AI operates on the principle of creating new content based on a given set of inputs, which can range from text prompts to images or even video sequences. At its core, generative AI harnesses sophisticated algorithms to generate novel material that adheres to the patterns and styles inherent in the provided input data.The process typically begins with a user submitting a prompt or input to the generative AI system. This input serves as the seed from which the AI generates new content. Whether it’s generating essays, solving complex problems, or producing realistic yet entirely fictitious images and audio clips, generative AI algorithms excel at producing diverse and often surprising outputs.Initially, engaging with generative AI systems required specialized knowledge and technical expertise.
Developers had to navigate complex APIs and implement programs using languages such as Python to interact with these systems effectively. However, as the field has advanced, pioneers have introduced user-friendly technologies that streamline the user experience.One notable advancement is the development of natural language processing (NLP) techniques that enable users to communicate with generative AI systems using plain language. This allows individuals to describe their requests in a manner akin to conversing with another human, greatly simplifying the interaction process. As a result, accessing the capabilities of generative AI has become more intuitive and accessible to a broader range of users.
Furthermore, advancements in user experience design have led to the creation of intuitive interfaces that facilitate seamless interaction with generative AI systems. These interfaces abstract away the complexities of the underlying algorithms, allowing users to focus on expressing their creative ideas and receiving high-quality outputs in return.
In essence, generative AI operates as a creative partner, leveraging vast datasets and sophisticated algorithms to produce content that is both novel and contextually relevant. The use of generative AI extends beyond simple content creation, encompassing a wide range of applications such as data processing, 3D modeling, and product design. By understanding the mechanisms behind generative AI and its evolving interface technologies, users can harness its power to unlock new realms of creativity and innovation.
Generative adversarial networks
Generative Adversarial Networks (GANs) represent a revolutionary approach in the realm of generative AI, building on the foundational concepts of the diffusion model. Unlike traditional generative models, GANs employ a distinctive architecture consisting of two recurrent neural networks that operate in tandem to perform multiple tasks: the generator and the discriminator. As a type of generative AI model, GANs are notable for their unique method of training two neural networks in a competitive manner, which includes the generative model creating fake data samples and the discriminative model distinguishing between real and fake data.
1. Generator - Crafting False Samples:
The first component of two neural networks, the generator, is aptly named for its role in creating synthetic data. This neural network begins with random noise as a seed and progressively refines it to generate data that resembles the real dataset it was trained on. This iterative process involves the generator continuously adjusting its parameters to produce increasingly realistic samples, effectively simulating the intricacies and patterns found in the genuine data.
2. Discriminator - Distinguishing Real from Fake:
In parallel, the second neural network, known as the discriminator, is designed to distinguish between authentic and generated data. This network learns to differentiate between the real dataset and the synthetic samples labeled data generated by the generator. As the training progresses, the discriminator becomes more adept at identifying the nuances that distinguish real data from artificially created data.
3. Adversarial Training - A Dueling Dance:
The genius of GANs lies in their adversarial training mechanism. The generator and discriminator engage in a constant duel, each striving to outsmart the other. As the generator refines its ability to produce realistic data, the discriminator simultaneously hones its capacity to discern between real and generated samples. This dynamic interplay between creation and detection is what propels the model to achieve unprecedented levels of generative capability.
4. Convergence - Mastery in Synthesis:
Through this adversarial process, GANs eventually reach a state of equilibrium, where the generator becomes highly proficient at generating data that is indistinguishable from real samples, and the discriminator struggles to train AI models to differentiate between the two. This convergence signifies the model’s mastery in synthesizing data that captures the essential characteristics of the training dataset.
Generative Adversarial Networks have proven to be exceptionally powerful across various domains, from image and video synthesis to text generation. Their ability to learn and mimic complex data distributions has propelled GANs to the forefront of generative AI, opening new frontiers in creative applications, and artistic endeavors, and even addressing challenges in data augmentation and synthesis for machine learning tasks. As GAN technology continues to evolve, the potential for groundbreaking advancements in generative AI remains virtually limitless.
Generative modeling
Generative modeling operates on a fundamentally different principle compared to discriminative modeling. While discriminative algorithms predict features based on given labels, generative model algorithms work in reverse, focusing on understanding the underlying relationship between features and labels. Rather than merely discerning boundaries between classes, generative models aim to capture the joint probability distribution of both the input features (X) and the corresponding labels (Y).
Mathematically, generative modeling involves estimating the probability of observing a particular combination of features and labels occurring together. By modeling the joint distribution of X and Y, generative algorithms gain a deeper understanding of the underlying data-generating process. This approach allows generative models to generate new samples that resemble the original data distribution, providing insights into the inherent structure and patterns within the synthetic data generation itself.
Generative modeling is particularly valuable in scenarios where understanding the relationship between different classes or categories is essential. For example, in image classification tasks, generative models can not only distinguish between different objects or animals but also generate new samples that belong to a specific class. This capability enables generative models to answer questions about the characteristics and features associated with particular classes, such as differentiating between a cat and other animals or generating realistic images of cats based on learned class distributions.
By focusing on the joint distribution of features and labels, generative modeling offers a holistic perspective on the underlying data, allowing for more nuanced analysis and synthesis of information. Whether in image generation, natural language processing, or other domains, generative models play a crucial role in uncovering the underlying structure of complex datasets and generating new insights that drive innovation and discovery.
Diffusion models represent an innovative class of generative models that enhance the process of creating new data. These models operate by iteratively applying controlled random changes to an initial data sample, gradually forming new samples that are coherent and high-quality. The iterative nature of diffusion models allows for a more nuanced control over the generation process, significantly reducing training time and improving the overall quality of the generated models. This makes diffusion models particularly suited for a wide range of generative AI applications, offering substantial benefits in terms of efficiency and versatility.
Here are the most popular generative AI applications
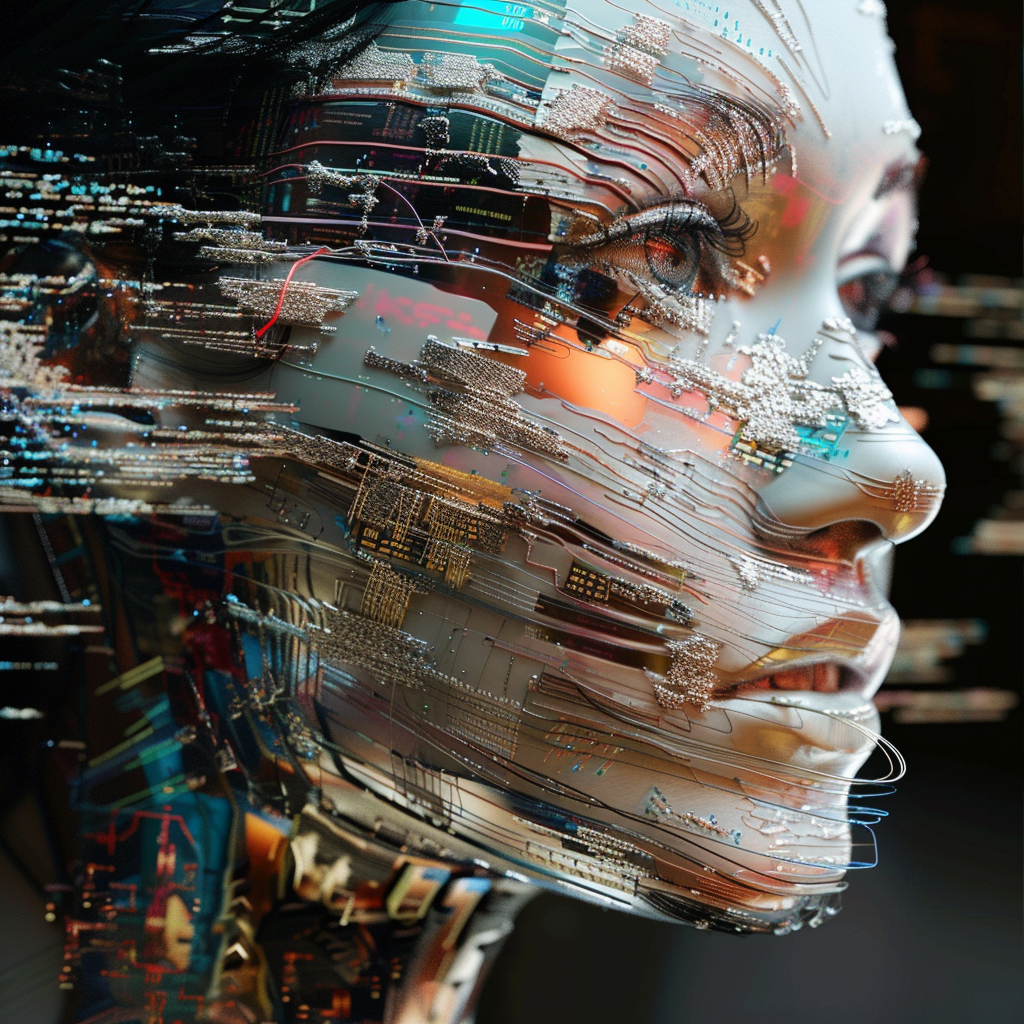
The advent of many generative AI models has significantly broadened the scope and enhanced the capabilities of generative AI across various sectors, including transportation and entertainment. These models, ranging from open-source initiatives to highly sophisticated systems, are pivotal in driving innovation and improving efficiencies in numerous applications:
1. Autonomous Vehicle Simulation and Training:
Generative AI is being used to simulate various driving scenarios to train and validate autonomous vehicle systems. By generating realistic virtual environments and scenarios, AI can refine the algorithms driving self-driving cars, making them safer and more adept at navigating real-world conditions.
2. Traffic Optimization and Prediction:
Generative AI algorithms analyze vast amounts of traffic data to predict congestion patterns and optimize traffic flow in urban areas. By generating predictive models based on historical data, transportation authorities can implement more efficient traffic management strategies, reducing commute times and alleviating congestion.
3. Personalized Entertainment Recommendations:
In entertainment, generative AI revolutionizes content recommendations. By analyzing user preferences and historical data, AI generates personalized recommendations tailored to individual tastes, enhancing the entertainment experience with content aligned to users’ interests.
4. Virtual Reality and Immersive Experiences:
Generative AI creates immersive virtual reality (VR) experiences by generating realistic environments, characters, and interactions. This technology enhances the sense of presence and realism in VR applications, providing users with immersive experiences ranging from simulated adventures to virtual training simulations.
5. Interactive Storytelling and Gaming:
Generative AI transforms interactive storytelling and gaming by dynamically generating content based on player actions. From procedurally generated game worlds to AI-generated dialogue and narratives, this technology creates dynamic gaming experiences that adapt to player choices, fostering engagement and replayability.
6. Music and Art Generation:
In the realm of creativity, generative AI empowers artists and musicians to explore new expressions. By analyzing artistic and musical data, AI generates original compositions, artworks, and designs, democratizing creativity and inspiring new forms of expression.
7. Customer Service Automation:
Generative AI is increasingly being employed in customer service automation, where it powers chatbots and virtual assistants. By generating human-like responses to customer queries and providing personalized assistance, AI-driven chatbots enhance customer service experiences, reducing response times and improving satisfaction levels.
These examples illustrate the diverse applications of generative AI across transportation, entertainment, and customer service automation, highlighting its potential to transform industries and enhance human experiences.
What are some generative models for natural language processing?
Among the most useful generative models for natural language processing (NLP) is the Transformer model, particularly exemplified by OpenAI's GPT (Generative Pre-trained Transformer) series. GPT models utilize a powerful architecture based on self-attention mechanisms, enabling them to generate coherent and contextually relevant text based on given prompts.
The key innovation of Transformer-based generative language models also lies in their ability to capture long-range dependencies and contextual information within the input text. By attending to all tokens simultaneously through self-attention mechanisms, these models excel at understanding and generating language in a manner that closely mimics human cognition.
Furthermore, GPT models leverage large-scale pre-training on diverse text corpora, allowing them to acquire broad linguistic knowledge and semantic understanding. This pre-training phase enables the models to generate high-quality text across a whole language model and a wide range of tasks and domains, making them invaluable tools for various NLP applications such as text generation, summarization, translation, and question-answering.
Overall, Transformer-based generative models, particularly exemplified large language models used by the GPT series, represent a significant advancement in natural language processing, offering unparalleled capabilities in text generation and understanding. Their versatility, accuracy, and scalability make them indispensable assets for researchers, developers, and practitioners seeking to harness the power of generative AI for language-based tasks.
What are the benefits of Generative AI?
Generative Artificial Intelligence (AI) offers a plethora of advantages across various industries, revolutionizing processes and unlocking new avenues for innovation. Here are several potential benefits of integrating generative AI into workflows:
1. Content Generation Automation:
One of the primary advantages of generative AI is its ability to automate the generation of new content. Whether it's text, images, or other forms of media, generative AI can streamline the content creation process by autonomously producing high-quality material based on predefined inputs or objectives. This automation not only accelerates production timelines but also frees up human resources for more strategic and creative tasks.
2. Simplified Content Interpretation:
Generative AI simplifies the interpretation of existing content by leveraging sophisticated algorithms to analyze and synthesize information. By automatically extracting insights, generating summaries, or translating content into different formats or languages, generative AI enhances the accessibility and usability of information, facilitating faster decision-making and knowledge dissemination.
3. Workflow Optimization:
Integrating generative AI into workflows has the potential to optimize and streamline processes across various industries. By automating repetitive tasks, generating custom solutions, or optimizing resource allocation, generative AI improves efficiency, reduces operational costs, and enhances overall productivity. This optimization allows organizations to focus on core competencies and strategic initiatives, driving competitive advantage and business growth.
4. Adaptation to Emerging Technologies:
Developers are actively exploring the integration of generative AI into workflows to adapt and harness emerging technologies effectively. By embracing generative AI-driven solutions, organizations can stay ahead of the curve, leveraging cutting-edge capabilities to address evolving challenges and capitalize on emerging opportunities. This adaptability enables businesses to maintain agility and resilience in dynamic and competitive markets.
5. Enhanced Creativity and Innovation:
Generative AI fosters creativity and innovation by augmenting human capabilities and expanding the realm of possibilities. By generating novel ideas, designs, or solutions, generative AI stimulates ideation, encourages experimentation, and catalyzes breakthrough innovations. This creative synergy between humans and AI unlocks new avenues for exploration and discovery, driving transformative change and disruptive innovation.
6. Personalization and Customization:
Generative AI enables personalized and customized experiences by tailoring content, products, and services to individual preferences and needs. By analyzing user data and generating personalized recommendations or solutions, generative AI enhances customer engagement, satisfaction, and loyalty. This personalization fosters deeper connections and fosters long-term relationships, driving business growth and success.
In summary, generative AI offers a multitude of benefits, ranging from automation and optimization to creativity and personalization. By harnessing the power of generative AI, organizations can unlock new opportunities, drive innovation, and gain a competitive edge in today's rapidly evolving digital landscape.
Use cases for generative AI, by industry
Generative AI technologies are often hailed as transformative tools with broad applications across various industries, akin to generating power or electricity due to their profound impact on entire business sectors. Unlike previous general-purpose technologies, which typically took decades to fully integrate into workflows, generative AI has the potential to rapidly revolutionize existing business processes, and unlock new opportunities for innovation and growth across diverse sectors. Let's explore some key industries and their potential use cases for generative AI:
1. Healthcare:
Generative AI can revolutionize healthcare by enabling personalized treatment plans, drug discovery, and medical image analysis. For example, generative models can generate synthetic medical images to augment limited datasets, assisting in training more accurate diagnostic algorithms and improving patient outcomes.
2. Finance:
In the finance industry, generative AI can mine proprietary data to be utilized for risk assessment, fraud detection, and algorithmic trading. Generative models can generate synthetic financial data to simulate market conditions, enabling better risk management strategies and more informed investment decisions.
3. Retail:
Retailers can leverage generative AI for personalized marketing, demand forecasting, and virtual try-on experiences. Generative models can generate realistic product images tailored to individual preferences, enhancing customer engagement and driving sales.
4. Entertainment:
Generative AI has vast applications in the entertainment industry, including content generation, virtual reality experiences, and personalized recommendations. For instance, generative models can create lifelike characters and environments for video games or generate personalized music playlists based on individual listening preferences.
5. Manufacturing:
In manufacturing, generative AI can optimize production processes, predictive maintenance, and product design. Generative models can generate 3D prototypes and simulate manufacturing workflows, reducing time-to-market and improving product quality.
6. Marketing and Advertising:
Generative AI can transform marketing and advertising through targeted messaging, content creation, and customer segmentation. For example, generative models can either generate content and ad copy tailored to specific audience segments or create personalized product recommendations based on past purchase behavior.
7. Education:
In education, generative AI can facilitate personalized learning experiences, automated grading, and content creation. Generative models can generate customized educational materials, adaptive learning paths, and virtual tutors to support student learning and engagement.
These are just a few examples of how generative AI can impact various industries by revolutionizing processes, unlocking new opportunities, and driving innovation. As generative AI continues to evolve, its potential to reshape entire industries and transform the way we work and live will only continue to grow.
Generative AI vs. predictive AI vs. conversational AI
Predictive AI, generative AI, deep learning method,s and conversational AI represent distinct approaches within the broader landscape of artificial intelligence, each with its unique capabilities and applications.
1. Predictive AI:
Predictive AI utilizes patterns in historical data to forecast future outcomes or trends. By analyzing large datasets, predictive AI algorithms can identify correlations and patterns that allow them to make informed predictions about future events. In organizations, predictive AI plays a crucial role in improving decision-making processes and creating data-driven strategies. By leveraging predictive insights, businesses can anticipate market trends, optimize resource allocation, and mitigate risks more effectively.
2. Generative AI:
Generative AI, on the other hand, focuses on creating new data or content based on existing patterns and structures within a given dataset. Unlike predictive AI, which forecasts outcomes, generative AI generates novel content that resembles the original raw data distribution. This content creation capability enables generative AI to produce text, images, music, and other forms of media autonomously. Generative AI has diverse applications, including text generation, image synthesis, and creative design.
3. Conversational AI:
Conversational AI enables AI applications to interact naturally with humans through conversation, typically in the form of chatbots or virtual assistants. By employing natural language processing (NLP) techniques, conversational AI systems analyze and understand human language inputs, allowing them to provide contextually relevant responses. Conversational AI enhances user engagement and customer service by enabling seamless interactions between humans and machines. Whether it's answering customer inquiries, providing product recommendations, or assisting with tasks, conversational AI facilitates intuitive and efficient communication.
In summary, while predictive AI focuses on forecasting future outcomes, generative AI creates new content, and conversational AI enables natural interactions between humans and machines through conversation. Each of these AI approaches offers unique benefits and applications, contributing to the advancement of technology and the enhancement of various aspects of our lives and businesses.
What are the limitations of generative AI?
While generative AI holds immense promise, its early applications also highlight several limitations inherent in the technology. These limitations stem from various factors, including the specific approaches used to implement generative AI applications and the complexity of the tasks at hand.
1. Quality and Fidelity:
One of the primary limitations of generative AI is the quality and fidelity of the generated content. While generative models have made significant strides in producing realistic outputs, they may still struggle with generating high-quality content consistently, especially in complex domains such as natural language or image synthesis.
2. Data Dependence:
Generative AI models heavily rely on the data they are trained on. Limited or biased training data can lead to the generation of inaccurate or biased outputs. Moreover, generative models may struggle with generating content outside the scope of their training or analyze complex data well, limiting their adaptability and generalization capabilities.
3. Computational Resources:
Training and deploying generative AI models often require substantial computational resources, including powerful hardware and extensive training time. This can pose challenges for smaller organizations or individuals with limited access to such resources, hindering widespread adoption and accessibility of generative AI technology.
4. Interpretability and Explainability:
Understanding how and why generative AI models generate specific outputs can be challenging. The black-box nature of some generative models makes it difficult to interpret their decisions, raising concerns about transparency, accountability, and trustworthiness, particularly in critical applications such as healthcare or finance.
5. Ethical and Societal Implications:
Generative AI raises ethical and societal implications related to issues such as misinformation, manipulation, and privacy. The potential for malicious actors to misuse generative AI technology for spreading fake news, generating deepfakes, or infringing on individuals' privacy underscores the importance of responsible development and deployment practices.
In summary, while generative AI holds immense potential, it is essential to recognize and address its limitations to ensure responsible and effective use of such models. By addressing challenges related to quality, data dependence, computational resources, interpretability, and ethical considerations, we can work towards harnessing the full benefits of generative AI while mitigating potential risks and drawbacks.
Conclusion
In conclusion, Generative AI stands at the forefront of technological innovation, poised to redefine the boundaries of human creativity and ingenuity. Through its ability to autonomously generate new content, whether in the form of text, images, music, or beyond, Generative AI promises to revolutionize industries, enhance user experiences, and drive unprecedented levels of innovation.
As we've explored throughout this article, Generative AI represents a paradigm shift in the field of artificial intelligence, enabling machines to not only mimic but also augment human intelligence in remarkable ways. From personalized entertainment recommendations to transformative applications in healthcare, finance, and beyond, the potential of Generative AI knows no bounds.
However, as with any powerful technology, Generative AI also raises important ethical, societal, and existential questions. As we continue to harness the capabilities of Generative AI, we must do so responsibly, ensuring that the benefits are equitably distributed and that potential risks are carefully managed.
Ultimately, Generative AI holds the promise of unlocking new realms of creativity, innovation, and discovery, shaping a future where the boundaries between human and machine intelligence blur evermore. By embracing this transformative technology with foresight, diligence, and a commitment to ethical principles, we can unlock its full potential to enrich lives, empower industries, and propel humanity forward into a new era of possibility and progress.