The Future of Customer Support: Trends in 2025
The customer support landscape is undergoing a revolutionary transformation, with artificial intelligence at its core and heightened customer expectations driving innovation at unprecedented rates. By the end of 2025, AI is projected to handle 95% of all customer interactions, fundamentally reshaping business operations and customer relationships. This comprehensive report explores the major trends reshaping customer support, examining how AI-powered solutions, personalization strategies, and omnichannel approaches are creating more efficient, effective, and emotionally intelligent customer experiences across industries. (McKinsey) (Hybrid Advances Vol 7)
Introduction
Customer support stands at the precipice of its most significant transformation in decades. Driven by rapid advancements in artificial intelligence and shifting consumer expectations, the traditional support model is evolving into a sophisticated ecosystem where technology and human expertise converge. According to recent projections, global spending on customer experience technologies will exceed $641 billion by 2025, representing a compound annual growth rate of 18.3% since 2020.
The modern customer expects instant gratification, personalized service, and seamless interactions across multiple channels. These expectations have accelerated the adoption of AI-powered support solutions, with 85% of customer interactions expected to begin with self-service by 2025. While traditional metrics like response time remain important, companies are increasingly focusing on predictive service models that anticipate customer needs before they arise.
The COVID-19 pandemic served as a catalyst for digital transformation, compressing years of technological adoption into months. This acceleration has permanently altered the customer support landscape, pushing organizations to embrace automation, remote service capabilities, and data-driven personalization at unprecedented rates.
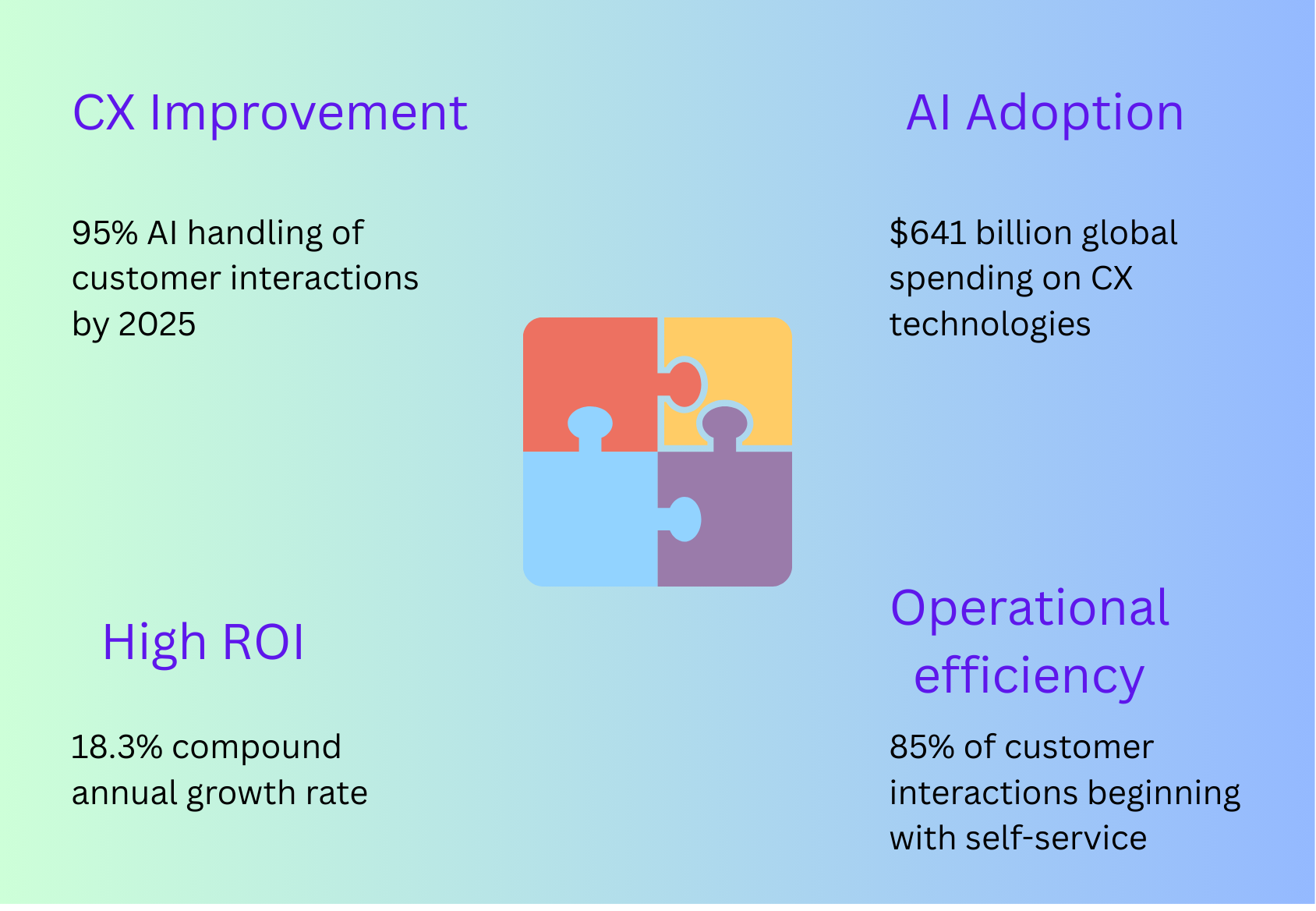
Historical Context and Evolution
Customer support has evolved dramatically over the past decades—from telephone-only call centers to multi-channel contact centers, and now to AI-powered experience hubs. This evolution reflects broader technological and social changes, with each iteration bringing greater efficiency, scalability, and personalization capabilities.
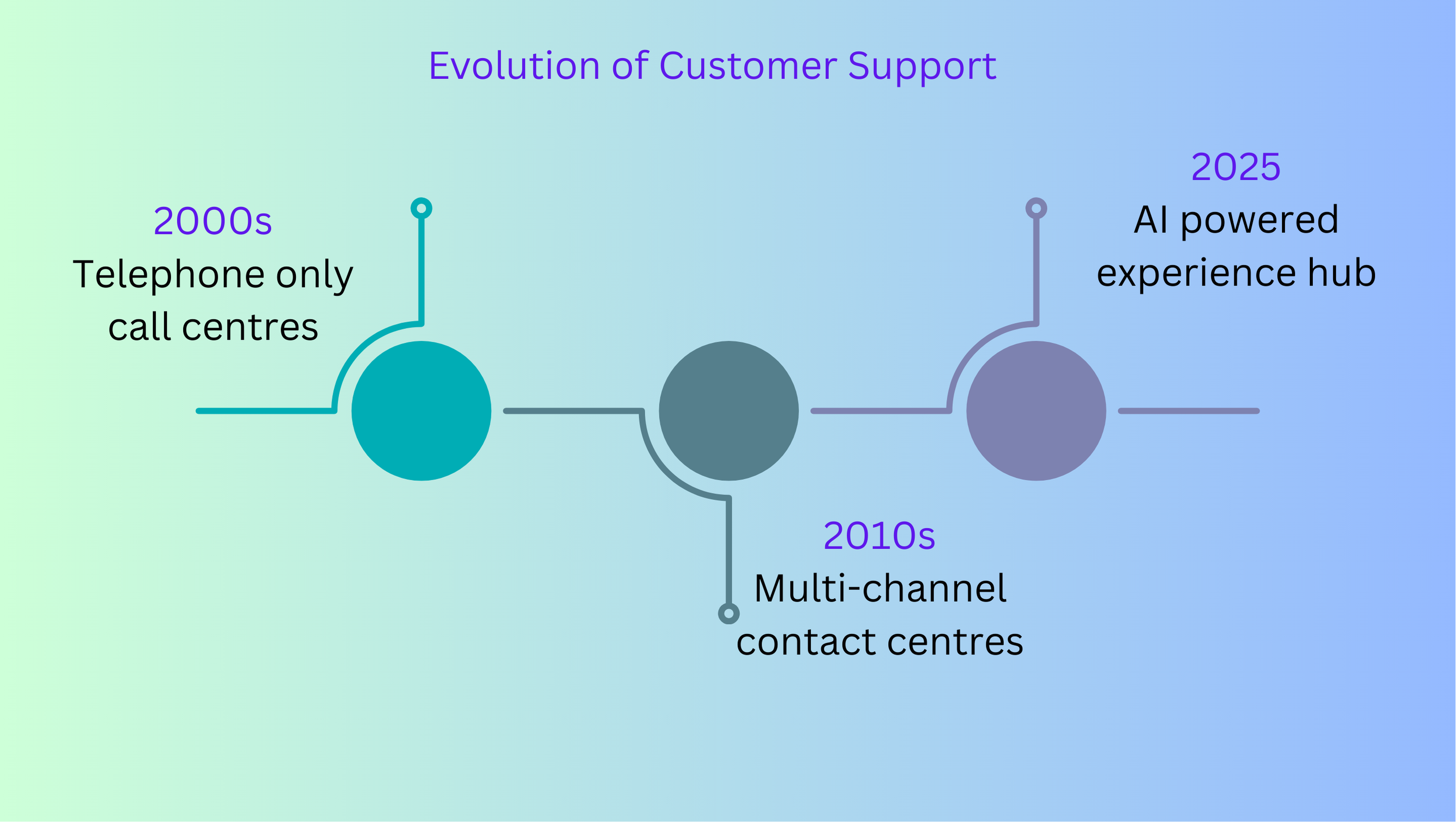
As we approach 2025, the integration of advanced AI capabilities represents the next evolutionary leap, fundamentally changing how businesses interact with customers while redefining roles for human support agents. This transformation is not merely technological but represents a paradigm shift in how customer relationships are managed and nurtured.
AI-Driven Automation and Efficiency
Automated Query Resolution
Artificial intelligence is revolutionizing query resolution through increasingly sophisticated chatbots and virtual assistants. By 2025, the global chatbot market is projected to exceed $1.34 billion, with enterprise adoption rates reaching 80% across major industries. These AI solutions offer compelling advantages: they can resolve up to 80% of routine queries without human intervention, provide continuous 24/7 support regardless of time zones, and reduce operational costs by up to 30%. (McKinsey)
The technological capabilities of these systems have advanced significantly, moving beyond simple rule-based interactions to conversational AI that understands context, remembers previous interactions, and learns from each engagement. Natural Language Processing (NLP) improvements have increased accuracy rates from 65% in 2020 to a projected 95% understanding by 2025, making interactions virtually indistinguishable from human conversations. (McKinsey)
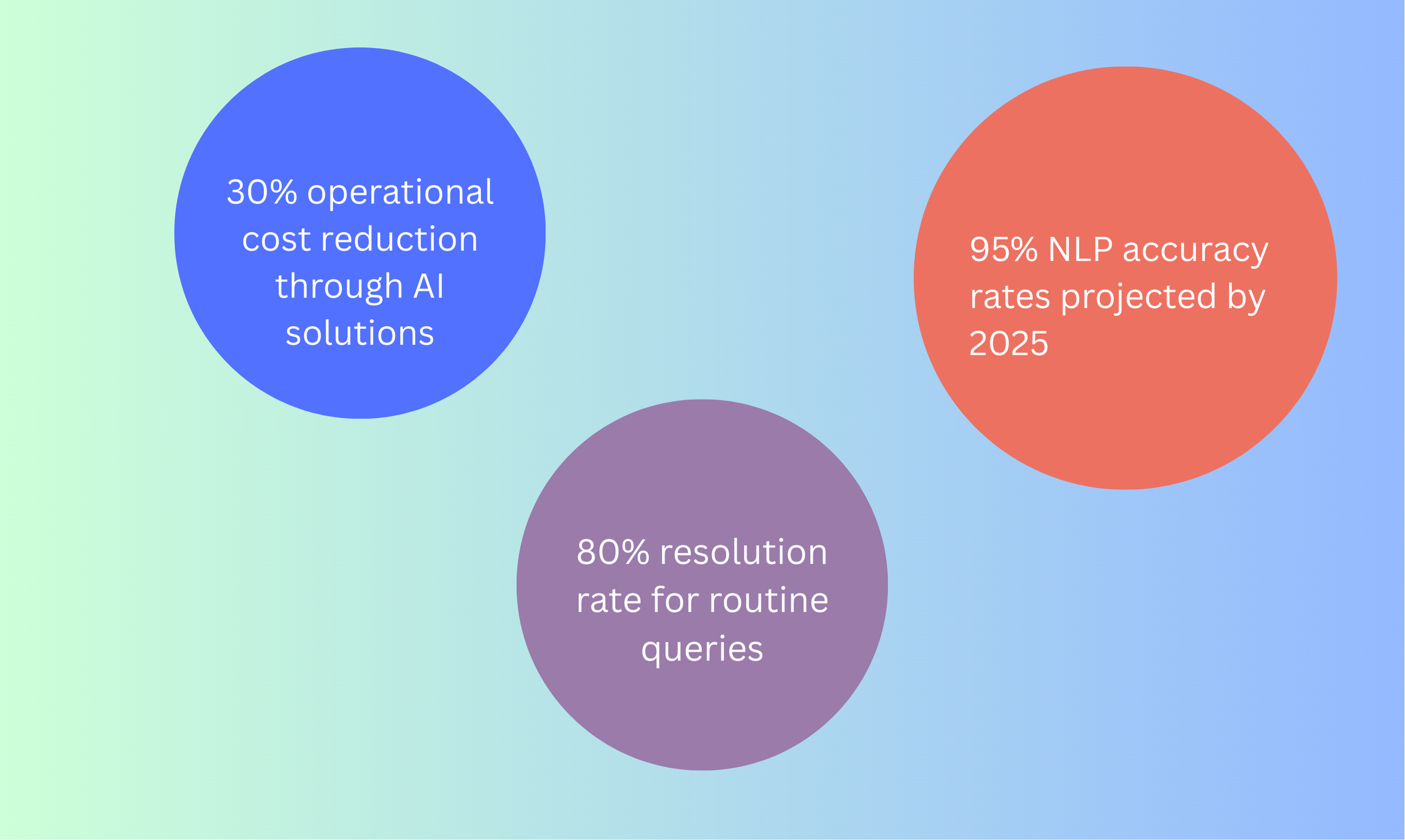
Industry-specific applications demonstrate the versatility of these solutions:
In retail, AI chatbots process return requests, track orders, and provide product recommendations with 78% satisfaction rates.
Healthcare providers deploy virtual assistants for appointment scheduling, medication reminders, and preliminary symptom assessment, reducing administrative workload by 35%.
Financial institutions utilize conversational AI for account inquiries, transaction verification, and basic financial advice, handling over 60% of routine banking questions.
Real-world implementations illustrate the dramatic impact: Bank of America's virtual assistant Erica now handles over 15 million client requests monthly, while H&M's shopping assistant has increased conversion rates by 30% when interacting with customers.
AI-Powered Self-Service
Customer preferences have shifted dramatically toward self-service solutions, with 76% of consumers now preferring to solve issues independently before contacting live support. This preference has driven the development of increasingly sophisticated self-service platforms powered by AI technologies.
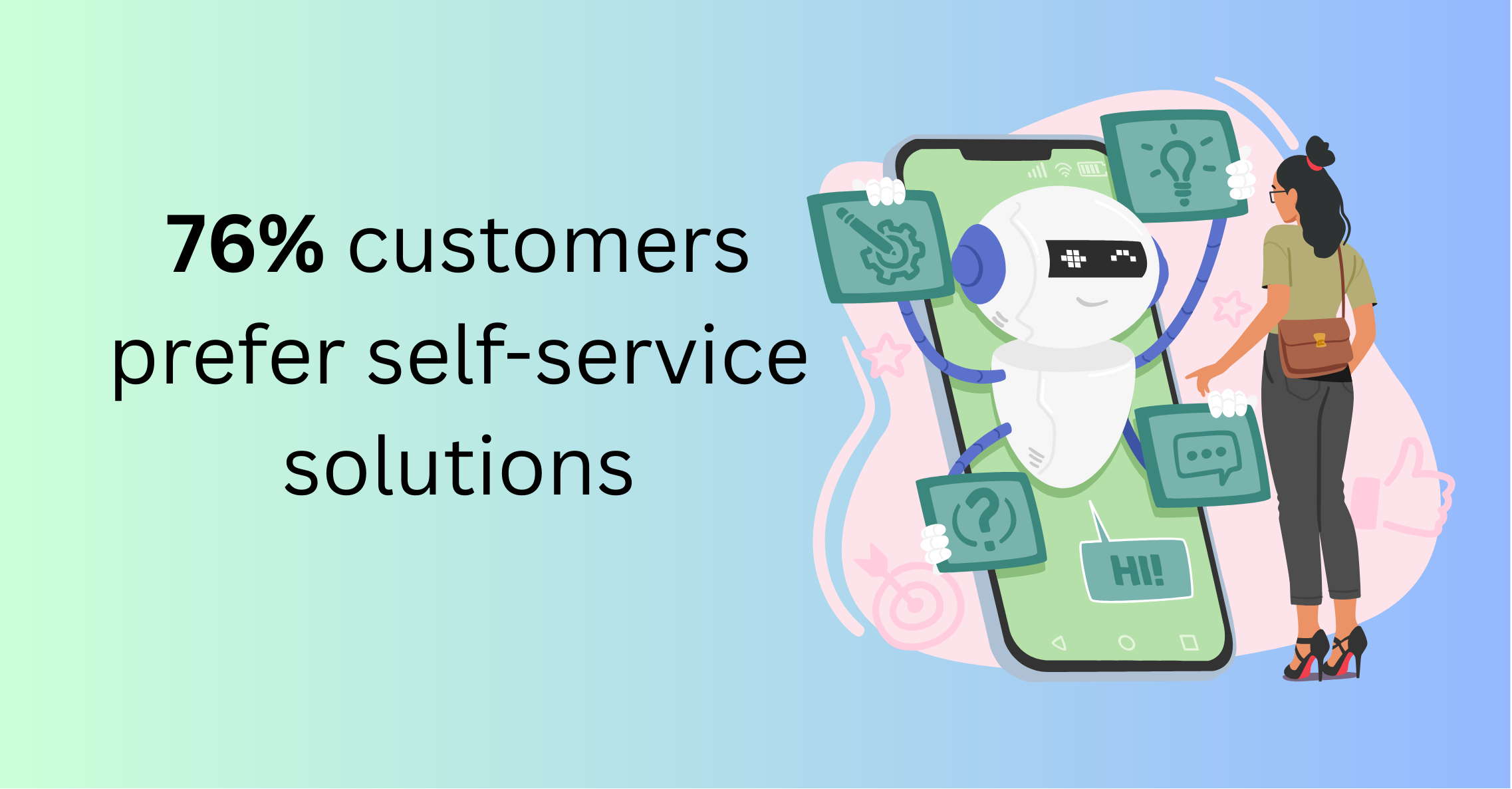
Modern self-service ecosystems incorporate several key components:
Dynamic knowledge bases that continuously update based on customer interactions, support tickets, and emerging issues.
Intelligent search algorithms that understand natural language queries and predict user intent with up to 87% accuracy.
Interactive troubleshooting tools that walk customers through complex procedures using decision trees optimized by machine learning.
Visual support options including AI-annotated screenshots, tutorial videos, and augmented reality overlays for physical products.
The integration of emerging technologies has further enhanced self-service capabilities:
Augmented Reality (AR) applications allow customers to visualize products in their environment or receive visual guidance for assembly and troubleshooting.
Voice-activated assistants provide hands-free support for complex procedures, with 40% of self-service interactions expected to be voice-initiated by 2025.
IoT device integration enables proactive self-service, with connected products automatically detecting potential issues and directing users to relevant solutions.
Companies implementing AI-powered self-service have reported significant benefits, including 35% reductions in support ticket volume, 28% improvements in customer satisfaction scores, and 45% decreases in resolution time for common issues (McKinsey).
Hyper-Personalization with Predictive Analytics
Data-Driven Personalization
Artificial intelligence has transformed personalization from a marketing nicety to a customer support imperative. By analyzing vast quantities of behavioral data, companies can now tailor interactions at the individual level with unprecedented precision. Organizations effectively leveraging AI-driven personalization report revenue increases of up to 40% and customer satisfaction improvements of 38%.
The technological foundation for this personalization relies on several data collection methodologies:
Cross-channel behavior tracking that creates unified customer profiles across web, mobile, in-store, and support interactions.
Natural language processing that analyzes support conversations to identify preferences, frustrations, and unmet needs.
Predictive modeling that anticipates future customer behaviors based on historical patterns and similar customer cohorts.
Real-time decision engines that dynamically adjust recommendations and support approaches during live interactions.
Regulatory considerations have become increasingly important in this data-rich environment. Successful personalization strategies now incorporate privacy-by-design principles, comply with regulations like GDPR and CCPA, and provide transparent opt-in mechanisms that build customer trust rather than eroding it. (McKinsey)
Industry leaders demonstrating personalization excellence include:
Streaming services that recommend support resources based on viewing history and common issues with specific content.
Financial institutions that provide personalized financial advice based on spending patterns, life events, and financial goals.
Healthcare providers tailor patient support resources according to medical history, treatment plans, and communication preferences.
Implementation requires sophisticated technology stacks incorporating customer data platforms (CDPs), machine learning capabilities, and real-time analytics engines that can process information and deliver personalized experiences without perceptible delays.
AI-Driven Emotional Intelligence
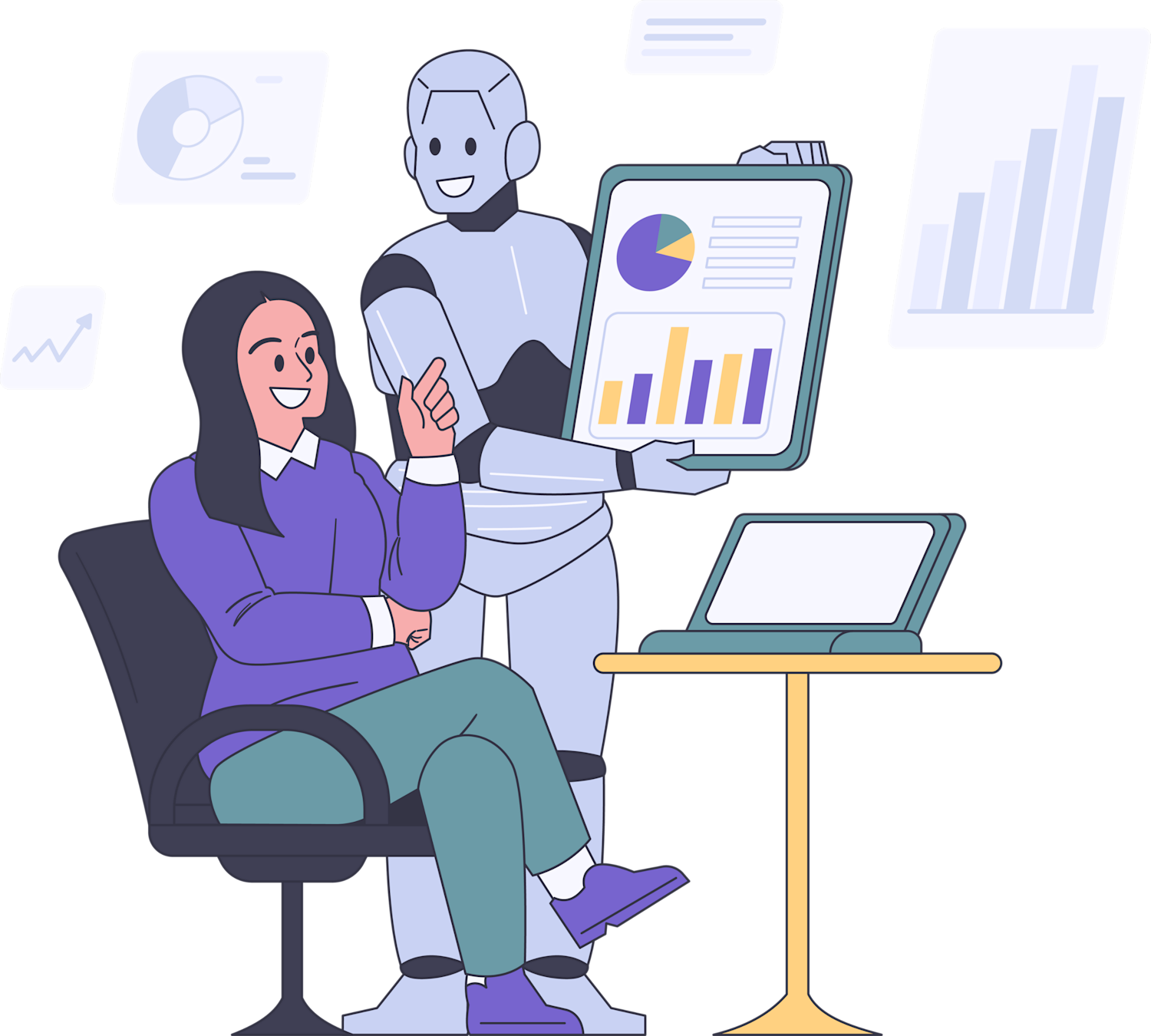
The emotional intelligence capabilities of AI systems represent perhaps the most profound advancement in customer support technology. By 2025, the emotional AI market is projected to reach $91.67 billion as businesses recognize the critical importance of empathy in customer interactions.
Advanced emotional AI systems incorporate multiple technologies:
Sentiment analysis algorithms that detect frustration, satisfaction, confusion, or urgency in written communications with 78% accuracy.
Voice pattern recognition that identifies emotional states through tone, pace, and pitch variations during phone interactions.
Facial expression analysis for video support calls that recognizes subtle emotional cues and adjusts responses accordingly.
Behavioral pattern recognition that identifies emotional states based on clicking patterns, typing speed, and interaction hesitations.
Industry applications demonstrate diverse implementations:
Mental health support platforms that adjust therapeutic approaches based on detected emotional states.
Luxury brand concierge services that calibrate tone and offerings according to customer mood and preferences.
Insurance claim processors that identify emotional distress during difficult claims and route to specially trained human agents.
Despite impressive advances, significant challenges remain in emotional AI deployment:
Cross-cultural emotional expression variations require region-specific training models.
Ethical concerns regarding emotional manipulation and privacy must be addressed through transparent policies.
Technical limitations still exist in detecting subtle emotional nuances, with accuracy rates dropping in ambiguous situations.
Research continues to advance the field, with developments in multimodal emotional detection combining text, voice, and visual cues to create more accurate emotional profiles that support agents can use to provide truly empathetic service. (Gartner)
Omnichannel AI Integration
Seamless Customer Journeys
Today's customers engage with brands across an average of nine different channels, expecting consistent, contextualized experiences regardless of touchpoint. AI technologies serve as the connective tissue that unifies these interactions, creating coherent customer journeys rather than fragmented encounters.
The integration challenges in creating truly seamless experiences are substantial:
Data silos between departments (marketing, sales, support) often create incomplete customer views.
Legacy systems lack interoperability with modern AI platforms, requiring complex integration layers.
Real-time data synchronization across channels demands a sophisticated architecture.
Complex customer journeys can involve dozens of touchpoints that must be mapped and optimized.
Successful implementations share common elements:
Unified customer data platforms that centralize information from all interaction points.
AI-driven journey orchestration tools that manage transitions between channels.
Real-time decision-making engines that personalize experiences based on journey context.
Predictive next-best-action recommendations that guide customers toward optimal resolutions.
Companies that effectively implement AI-powered omnichannel strategies see remarkable results, including 91% higher customer retention rates, a 23% increase in revenue, and a 33% reduction in support costs. These benefits accrue from several factors:
Elimination of repetitive information gathering across channels.
Contextually aware support that builds on previous interactions.
Proactive intervention at critical journey points before problems escalate.
Consistent brand voice and personalization across all touchpoints.
The backend systems required for omnichannel AI implementation typically include API layers for service integration, real-time event processing capabilities, sophisticated identity resolution technologies, and advanced analytics platforms that continuously optimize journey performance.
Voice and Speech Analytics
Voice-enabled AI represents one of the fastest-growing segments in customer support technology, with 50% of all searches expected to be voice-based by 2025. Advanced speech analytics has transformed voice from a single-dimension channel to a rich data source that provides deeper customer understanding.
Key technological advances driving this evolution include:
Natural language understanding that comprehends complex queries with contextual awareness.
Speech-to-text accuracy rates exceeding 97%, even with diverse accents and background noise.
Voice biometrics provides secure authentication without friction.
Emotion detection through voice pattern analysis identifies customer satisfaction or frustration.
Applications extend far beyond basic voice assistants:
Call center voice analytics that reduce handle time by 60% while providing real-time agent coaching.
Healthcare applications that detect cognitive changes, stress levels, or medication adherence through voice pattern analysis.
Financial services use voice analysis for security verification and fraud detection.
The automotive industry is deploying sophisticated in-car voice assistants for hands-free support.
Despite rapid advancement, voice technology faces unique challenges:
Privacy concerns regarding ambient listening and recording practices.
Accessibility considerations for speech-impaired customers and diverse language support.
Environmental factors, like background noise that can impair recognition accuracy.
Cultural and regional speech variations require localized training models.
Future developments in voice AI include more sophisticated contextual understanding, improved emotional intelligence, multilingual capabilities, and tighter integration with visual interfaces for multimodal interactions. (Gartner)
AI in Customer Support Operations
AI-Augmented Human Agents
While automation handles increasing volumes of routine inquiries, human agents remain essential for complex problem-solving, emotional connection, and judgment-intensive scenarios. AI now serves as an intelligence amplifier for these human agents, dramatically improving their capabilities and job satisfaction.
Modern agent augmentation tools include:
Real-time knowledge recommendation systems that suggest relevant solutions as customers explain issues.
Conversation analysis tools that identify customer sentiment and suggest appropriate responses.
Automatic summarization of previous interactions across all channels.
Process automation for administrative tasks, allowing focus on actual customer needs.
Predictive issue classification that prepares agents before conversations begin.
Implementation requires thoughtful training approaches:
Technical training on AI tool functionality and limitations.
Soft skills development for scenarios requiring human judgment.
Collaboration techniques between AI systems and human agents.
Critical thinking for evaluating AI recommendations.
Organizations implementing AI augmentation report substantial benefits:
35% increase in first-contact resolution rates.
27% improvement in agent satisfaction scores.
40% reduction in average handle time for complex issues.
62% reduction in agent training time through AI-assisted onboarding.
Case studies demonstrate diverse approaches to human-AI collaboration. Financial services firm JPMorgan Chase deployed an AI system called COIN that reviews complex loan agreements in seconds rather than the 360,000 hours previously required by legal teams. Healthcare provider Kaiser Permanente uses AI to summarize patient history and suggest relevant questions for support staff, reducing call times by 28%.
Workforce Transformation
The integration of AI into customer support necessitates a fundamental rethinking of workforce skills, structure, and development. By 2025, 80% of customer support teams will use AI tools, creating both challenges and opportunities for the human workforce. (Gartner)
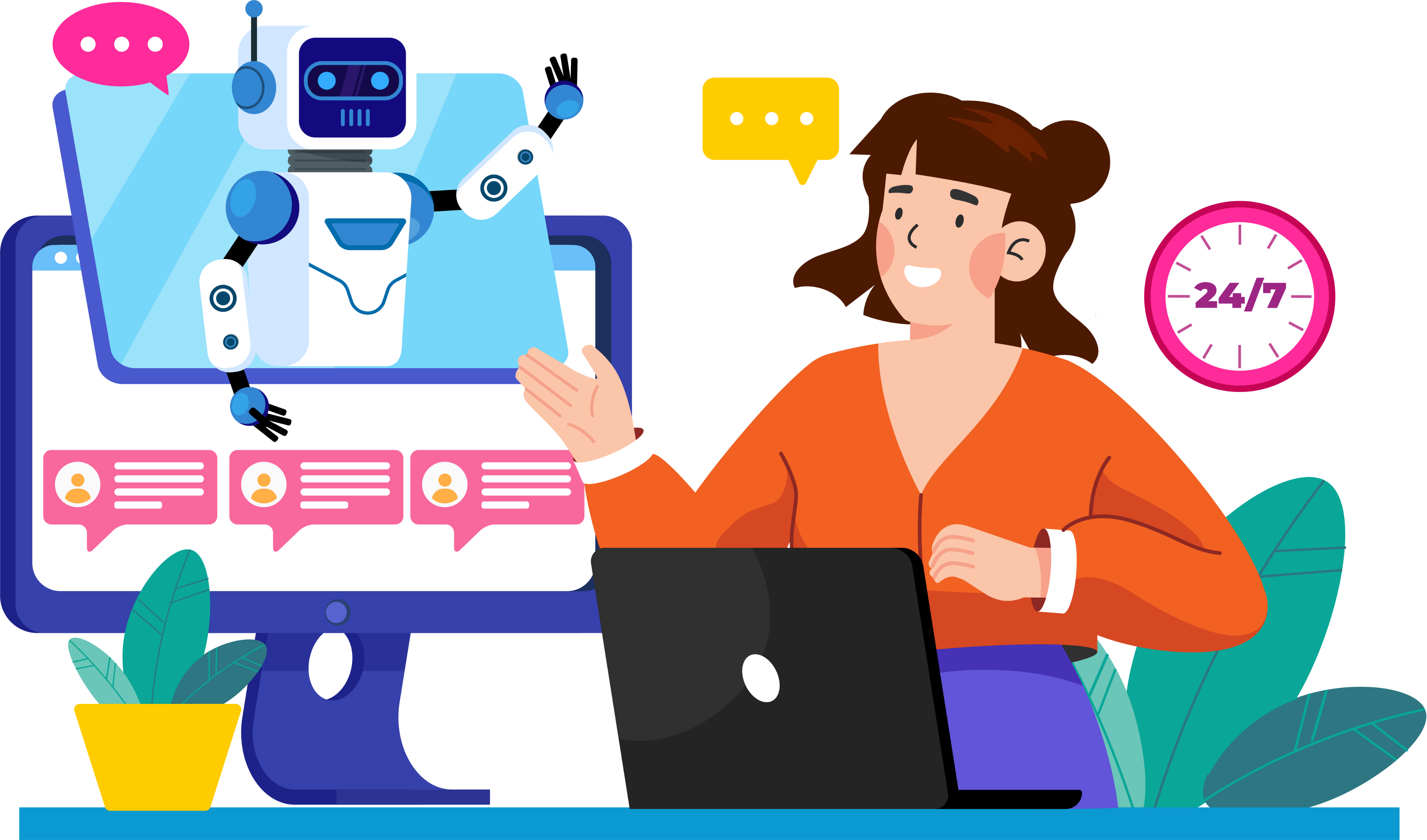
Essential skills for future support professionals include:
Complex problem-solving capabilities for issues that exceed AI capabilities.
Emotional intelligence and empathy that remains distinctively human.
Technical literacy to effectively collaborate with AI systems.
Adaptability and continuous learning mindsets as technologies evolve.
Strategic thinking to identify systemic issues beyond individual cases.
Organizational transformation requires structured change management approaches:
Clear communication about how AI will change roles rather than eliminate them.
Phased implementation that allows for adaptation and feedback.
Skills assessment and personalized development paths for existing staff.
Revised performance metrics that reflect new collaborative models.
Modified recruitment profiles emphasizing complementary human skills.
Training programs are evolving to support this transition:
Immersive simulations of human-AI collaboration scenarios.
Micro-learning modules delivered at the point of need.
Peer learning communities that share best practices.
Technical certifications in relevant AI platforms.
Cross-functional rotations that build broader business understanding.
The job market impact of these changes includes the emergence of new roles such as AI trainers, automation specialists, and customer journey architects, while traditional roles like basic tier-one support will continue to decline. Organizations that invest in reskilling rather than replacement report 3.5x higher retention rates and significantly higher customer satisfaction scores. (McKinsey)
Ethical Considerations and AI Governance
Addressing AI Bias
As AI systems increasingly make or influence decisions in customer support contexts, addressing bias has become a critical ethical and business imperative. Research indicates that biased AI systems can reduce customer trust by up to 78% and expose organizations to significant reputational and legal risks. (Twilio Segment)
AI bias manifests in multiple forms within customer support:
Demographic bias that provides different service levels based on gender, race, age, or location.
Statistical bias from unrepresentative training data that fails to account for diverse user scenarios.
Measurement bias where evaluation metrics favor certain customer groups over others.
Temporal bias where systems fail to adapt to changing customer behaviors and expectations.
Detecting and mitigating these biases requires robust methodologies:
Diverse training datasets that represent the full customer spectrum.
Regular algorithmic audits by independent third parties.
Fairness metrics incorporated into development and testing processes.
Adversarial testing specifically designed to identify potential biases.
Ongoing monitoring of real-world performance across customer segments.
Regulatory developments are accelerating in this space, with the EU's Artificial Intelligence Act and similar legislation establishing legal frameworks for AI fairness, transparency, and accountability. Organizations leading in this area implement governance structures that include ethics review boards, clear accountability for AI outcomes, and transparent remediation processes when biases are identified.
Data Privacy and Security
Customer support AI systems require vast quantities of personal data to function effectively, creating significant privacy and security considerations. Organizations must balance personalization benefits against privacy risks in an increasingly regulated environment.
The global regulatory landscape continues to evolve:
GDPR in Europe has established strict standards for consent and data minimization.
CCPA and similar state laws in the US have created a patchwork of requirements.
Industry-specific regulations like HIPAA add additional complexity for healthcare support.
Emerging global standards aim to harmonize requirements across jurisdictions.
Technical safeguards have become increasingly sophisticated:
Privacy-preserving AI techniques, including federated learning and differential privacy.
End-to-end encryption for sensitive customer communications.
Data minimization architectures that limit collection to essential information.
Automated retention policies that delete data when no longer needed.
Anonymization techniques that maintain analytical utility while protecting identities.
Customer trust represents perhaps the most critical consideration, with research showing:
73% of consumers prefer brands that transparently use AI for personalization.
82% are willing to share more data when privacy policies are clear and controls are robust.
65% have abandoned a company after a data breach or privacy concern.
91% believe companies should be held legally liable for data misuse.
The impact of security breaches extends beyond regulatory penalties to significant business costs, with the average cost of a data breach reaching $4.35 million in 2024. AI-based security measures can detect and mitigate fraud 50% faster than traditional methods, creating a virtuous cycle where AI helps protect the very data it requires to function effectively. (McKinsey)
Implementation Strategies and ROI
Assessing Organizational Readiness
Before embarking on advanced AI customer support initiatives, organizations must systematically evaluate their readiness across multiple dimensions. This assessment process helps identify gaps, establish priorities, and create realistic implementation timelines.
Key readiness factors include:
Data maturity: The quality, accessibility, and governance of customer data fundamentally determines AI capabilities.
Technical infrastructure: Existing systems must support real-time processing and integration requirements.
Organizational culture: Teams must be prepared to adapt workflows and embrace AI collaboration.
Talent availability: Internal skills or access to external expertise must be secured.
Customer expectations: Target audiences vary in their receptiveness to AI-driven support.
Assessment methodologies typically employ:
Capability maturity models that benchmark current states against industry standards.
Gap analysis tools identifying specific deficiencies requiring remediation.
ROI modeling to prioritize investments with highest potential returns.
Pilot programs testing limited implementations before broader deployment.
Industry benchmarking provides context for readiness evaluations, with sectors like technology and financial services typically showing higher maturity than healthcare or government services. This benchmarking helps establish realistic timelines and expectations based on comparable organizations. (Mckinsey)
Implementation RoadmapSuccessful AI customer support implementations follow structured roadmaps that balance quick wins with long-term transformation. A phased approach allows organizations to demonstrate value while building capabilities over time.
Effective implementation typically follows this sequence:
Foundation Building (3-6 months)
Data cleanup and integration
Governance framework establishment
Initial team training and change management
Vendor selection and partnership development
Pilot Deployment (2-3 months)
Limited-scope implementations in controlled environments
Performance baseline establishment
Feedback collection and system refinement
ROI validation
Scaled Implementation (6-12 months)
Phased rollout across channels and departments
Comprehensive staff training programs
Process re-engineering to leverage AI capabilities
Integration with existing customer experience infrastructure
Continuous Optimization (Ongoing)
Performance monitoring and enhancement
Model retraining with new data
Expansion to additional use cases
Advanced capability development
Resource allocation considerations include:
Budget distribution across technology, training, and change management
Balancing internal development with vendor solutions
Appropriate staffing for implementation and ongoing management
Executive sponsorship and cross-functional governance
Organizations that follow structured implementation approaches report 65% higher success rates and 40% faster time-to-value compared to ad-hoc implementations.
Measuring ROI
Demonstrating the return on AI customer support investments requires comprehensive measurement frameworks that capture both direct financial impacts and indirect benefits.
Key performance indicators typically include:
Efficiency metrics: Cost per interaction, handle time, first-contact resolution
Revenue metrics: Conversion rate, upsell success, customer lifetime value
Experience metrics: Customer satisfaction, Net Promoter Score, effort score
Operational metrics: Agent turnover, training time, system uptime
Calculation methodologies must account for both easily quantifiable benefits and more complex value drivers:
Direct cost savings from the automation of routine inquiries
Increased revenue from improved customer experience and retention
Reduced opportunity costs from faster issue resolution
Risk mitigation value from improved compliance and consistency
Case studies demonstrate compelling returns across industries:
A telecommunications provider achieved 340% ROI over three years through reduced handle time and improved first-contact resolution.
An e-commerce retailer increased conversion rates by 28% through AI-powered personalized support.
A healthcare system reduced operational costs by $3.8 million annually while improving patient satisfaction scores.
Analysis of successful implementations indicates that organizations typically see positive ROI within 12-18 months, with mature implementations delivering 3-5x returns over three years. (KPMG)
Future Outlook Beyond 2025
Emerging Technologies
While current AI applications are transforming customer support, emerging technologies promise even more profound changes beyond 2025. These developments will further blur the lines between human and artificial intelligence while creating entirely new support paradigms.
Quantum computing represents perhaps the most significant long-term development, with potential impacts including:
Exponentially more powerful machine learning models that understand context and nuance at near-human levels.
Real-time processing of massive multimodal datasets that enable truly predictive support.
Complex simulation capabilities that test experience designs across countless scenarios.
Encryption-breaking potential that will require fundamental security rethinking.
Brain-computer interfaces (BCIs) and neural technologies, while still emergent, suggest future interfaces where:
Customers communicate needs through thought patterns rather than explicit requests.
Support agents receive information through direct neural feeds rather than screens.
Emotional states are detected and responded to with unprecedented accuracy.
Training occurs through direct neural implantation rather than traditional learning.
Advanced language models continue their rapid evolution with capabilities including:
Perfect multilingual support without translation artifacts.
Complete understanding of cultural nuances and implicit communication.
Generation of creative solutions beyond pre-programmed responses.
Dynamic personality adaptation to match individual customer preferences.
While some of these technologies remain speculative, organizations should establish horizon scanning processes to monitor developments and assess potential competitive impacts.
Shifting Customer Expectations
The customer of 2025 and beyond will bring fundamentally different expectations shaped by technological immersion, demographic shifts, and changing cultural norms.Generation Z and Alpha consumers, who will represent over 40% of the customer base by 2028, demonstrate distinct preferences:
Video-first communication rather than text or voice.
Expectation of immediate, 24/7 resolution via preferred channels.
Higher privacy awareness combined with the willingness to share data for clear benefits.
Strong preference for brands aligned with personal values and social concerns.
Accessibility considerations are becoming central rather than peripheral:
Universal design principles applied to all customer interactions.
Support for diverse physical, cognitive, and neurological needs.
Multimodal interaction options providing true choice rather than limited alternatives.
Real-time adaptation to individual communication preferences and capabilities.
Global market differences require increasingly nuanced approaches:
Varying technology adoption rates requiring hybrid support models.
Cultural expectations around service delivery and communication styles.
Regional regulatory frameworks necessitating localized governance.
Economic disparities influencing access to advanced support channels.
Organizations that anticipate these shifts can create a competitive advantage through forward-looking experience design, while those that remain reactive will face increasingly challenging customer acquisition and retention.
Conclusion
The customer support landscape of 2025 represents a fundamental departure from traditional service models, with AI serving as both the foundation for efficiency and the enabler of unprecedented personalization. Organizations that successfully navigate this transformation will realize significant competitive advantages through reduced costs, enhanced customer loyalty, and sustainable revenue growth.
Several key principles emerge for organizations seeking to thrive in this new environment:
Human-AI collaboration must be viewed as a partnership that amplifies unique human capabilities rather than a replacement strategy.
Ethical considerations cannot be afterthoughts but must be integrated into the foundation of AI systems design and governance.
Data strategy serves as the critical enabler, with quality and integration determining ultimate capabilities.
Implementation approaches must balance technological possibilities with organizational readiness and change management realities.
Continuous evolution rather than point-in-time transformation will characterize successful programs.
The organizations that will excel are those that view customer support not merely as a cost center but as a strategic differentiator capable of creating sustainable competitive advantage. By embracing the AI-powered future while maintaining human connection, these companies will define new standards for customer experience in 2025 and beyond.
Book a personalized demo to leverage IrisAgent's human-like AI Agents and begin your journey toward the future of customer support today.