How does historical support data unlock support automation use cases?
Recent years have seen tremendous advancements in Conversational AI, where systems can understand user queries and respond in a way that simulates natural conversations. Furthermore, Generative AI is advancing Conversational AI by having the ability to generate tailored and contextual responses to user queries.
Given the promise of the emerging technologies, not to mention the hype around ChatGPT, Support organizations are re-evaluating their support automation use cases. Conversational AI can profoundly transform support automation with 60%+ automated responses that are accurate and human-like in response and eventually help CSAT and the business bottom line. While the technology has evolved, organizations often struggle with how best to start - i.e., identify use cases primed for automation. This article explains how analysis of historical support data can help accurately identify key use cases that can be automated.
Contents
What is Conversational AI for Support Automation
Getting Started - Understanding Historical Support Data
Organizing Historical Insights and Intent with Auto-tagging
Running a Successful Proof of Concept (POC)
Best practices of conversational AI for Customer Service
Conclusions
FAQs
What is Conversational AI for Support Automation?
Customer service and support functions across industries have greatly benefited from the evolution of Conversational AI technologies. Conversational AI for support automation primarily manifests itself in the following use cases,
Chatbots: Many companies utilize chatbots on their websites and mobile applications to provide immediate assistance to customers. For example, a bank might employ a chatbot to handle basic inquiries about account balances, transaction history, etc.
Intelligent Voice Agents: Voice assistants like Amazon's Alexa or Google Assistant are being integrated into customer service workflows. There are companies that specialize in building Intelligent Voice Agents for customer service as a specialty - IrisAgent, Omilia, Nuance, Avaamo, Yellow.ai, and Kore.ai are a few that have gained traction.
Agent Assistance: Call center and customer service agents can get access to relevant content and responses to customer queries using Conversational AI capabilities.
AI-Powered Knowledge Bases: Some customer service platforms use AI to develop and maintain extensive knowledge bases. These AI systems can retrieve relevant information to support customer queries, providing quick and accurate answers to a wide range of questions.
Getting Started - Understanding Historical Support Data
With the advancements in Conversational AI, support leaders are revisiting support automation use cases. While the technology promises to automate 60%+ of customer queries, one key challenge customer service leaders face is - How do you get started?
One of the most reliable ways to understand what to automate is to look at historical support data. Simply put, when you analyze historical data on customer queries, it helps you identify repeat patterns, topics, and intents that can easily uncover key use cases ripe for automation.
Following are some key sources of historical support data,
CRM Ticketing information - Most support organizations have some of the popular CRM systems such as Salesforce, Zendesk, intercom, Freshworks, ServiceNow and Microsoft Dynamics installed. These systems store customer queries in the form of tickets or cases. Analyzing historical tickets or case data sheds light on repeat queries.
Call Recordings in Contact Centers - Most contact center operations store call recordings of customer interactions. These recordings often can unveil repeat patterns for customer queries,
Agent Chat transcripts - For organizations that have implemented online chat support, chat transcripts can offer insights into repeat patterns and customer intent.
Search queries on Support portal - For organizations that have implemented support portals, customers often search for answers via natural language or keyword-based queries. Analyzing these queries sheds a lot of light on the type of questions customers typically ask.
Q&A or support forums - For organizations that have Quora-like enterprise customer communities, Q&A or support forums are excellent sources of common customer questions.
Organizing Historical Insights and Intent with Auto-tagging
With vast amounts of historical data - how does one organize the insights and intents uncovered? Thanks to AI-based auto-tagging capabilities, Support automation platforms such as IrisAgent have developed specialized algorithms that help classify content for keywords associated with root-cause analysis, correlations to existing issues, customer intent, and sentiment. Thus, all the insights and intents uncovered are very quickly summarized with auto-tagging: keywords that efficiently encapsulate the issue/topic as well as intents expressed by customers. Accuracy in tagging thus forms the starting point for support automation. With accurate tagging, it is easy to train your data to LLMs and configure conversational dialogs and workflows. Furthermore, auto-tagging can very quickly help organizations bucket automation use cases in the following three categories,
Full automation, i.e., no human intervention, Via bots, Intelligent Virtual Agents for Voice and digital support interactions. The frequency and recency of auto-tagging of content guide what should be fully automated. The IrisAgent platform specializes in auto-tagging, quickly highlights the top tags, and provides recommendations on what to automate to improve overall customer experience.
Agent Assist: This is where AI helps an agent address questions with potential answers in real time. Agent Assist is designed to aid the agent rather than take over full automation.
Workflows and alerts: This is the category where items not automated in the aforementioned steps are seamlessly handled via intelligent workflows that alert the right support representative of subject matter experts. IrisAgent platform allows for easy configuration of alerts and notifications to cross-functional teams.
Running a Successful Proof of Concept (POC) for customer support automation
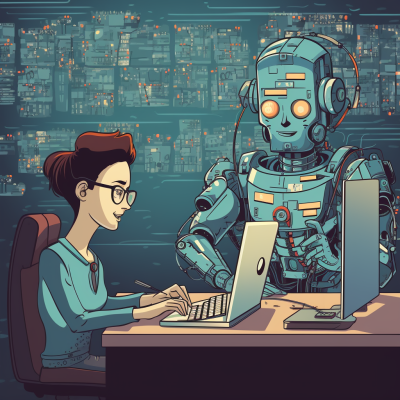
Large organizations often have complex workflows, compliance needs, and change management cadence that must be addressed before customer automation strategies can be released. This is a particular challenge for Conversational AI - wherein there is always the challenge of consumer backlash with over-automation, compliance needs to be adequately addressed, and changes to existing workflows and SLAs need to be considered. In these situations, running a Proof of Concept is a good way to verify the efficacy of the technology and the use cases being automated. IrisAgent offers Proof of Concept to validate use cases, and over the last 2+ years, we have learned a few good lessons. When running a Proof Of Concept, the following are a few best practices,
Start with a few key use cases: This is the most important. A POC is not a validation of an RFP or RFI questionnaire. Rather, it is a validation of key functionality of a platform as applied to your key use cases. Thus, identifying key use cases and running a POC against those limited sets of use cases is key to understanding whether IrisAgent or similar platforms are built to address your key automation scenarios.
Identify key success criteria/KPIs: The key to a POC success is identifying success criteria - such as the percentage of queries automated, the accuracy of responses, etc. Stick to a few that matter most. Also, we want to be realistic; 100% automation is aspirational to start with; we encourage a more realistic approach where the goal should be to improve upon what you have today.
Make sure cross-functional teams are aligned on success criteria: In large organizations, we often come across cross-functional teams and many personnel involved in decision-making. While finance may be interested in cost, support teams focus more on automation, and the CISO is more interested in security and compliance. The KPIs identified for the POC must be understood and agreed to by the cross-functional teams.
Access to historical support data and auto-tagging is a prerequisite - Most important in our view, especially when running IrisAgent POC. This should be rather obvious as well. To measure efficacy, access to historical data is a must, as it determines the level of automation that IrisAgent can deliver. Without access to historical data, the POC will not meet its true objective - understanding the full breadth of IrisAgent functionality.
Allow for adequate testing and iterative fine-tuning - While irisAgent is designed to work right out of the box, it allows for iterative fine-tuning of intent detection and automation for large organizations and complex deployments. IrisAgent AI specialists will often fine-tune the algorithms and tailor them to the specific needs of the POC use cases.
Conclusions
Conversational AI has gained significant traction in revolutionizing support automation. With technological advancements, support leaders are re-looking at automation strategies; however, getting started can often be challenging. IrisAgent has been helping organizations with automation strategies for the past 2+ years. The best way to do so is,
Understanding topics/questions and intent from historical data analysis
Unlocking the power of auto-tagging to enable automation with Conversational AI
Running effective Proof of Concept against key use cases
Following best practices that call for an iterative approach to maximize automation ROI
FAQs
What is Conversational AI?
Conversational AI refers to artificial intelligence (AI) that focuses on technology and systems capable of engaging in natural and human-like conversations with users. Key components that make up conversational AI include:
Natural Language Processing (NLP): NLP is the technology that enables machines to understand and interpret human language. It involves tasks like text parsing, sentiment analysis, and language understanding.
Speech Recognition: In applications that involve spoken language, speech recognition technology is used to convert spoken words into text, allowing the system to process and respond to voice commands.
Natural Language Generation (NLG): NLG is the process of generating human-like text or speech in response to user input. It's crucial for providing coherent and contextually relevant responses. The advent of LLMs and generative AI has driven significant advancements in this area.
Machine Learning: Machine learning algorithms are often employed to train conversational AI models using large datasets of human-generated conversations. These models learn to understand and generate human language.
Dialog Management: Dialog management systems help maintain a coherent and context-aware conversation. They decide what the system should say or do next based on the current conversation context.
Knowledge Base: Many conversational AI systems are integrated with a knowledge base that contains information that the AI can reference to answer questions accurately.
Why should organizations care?
Conversational AI, when done right, can significantly improve CSAT, increase call deflection rates, improve agent performance, and increase first-call resolution (FCR) rates. With these improvements, businesses can drive better financial performance - saving operational costs while improving customer retention and the top line.
What are the best sources for historical data?
CRM tickets, customer support call recordings, chat transcripts, support portal queries, and Q&A from online forums are excellent sources to tap into.
What is the benefit of auto-tagging?
Auto-tagging unlocks topics, questions, and intent that thus forms the basis of support automation use cases. Auto-tagging forms the foundation of the IrisAgent Support Automation platform. The better the auto-tagging, the better the automation efficiency.
What are the key aspects of running a successful POC?
Identifying a few key use cases, ensuring success criteria are defined, allowing for auto-tagging and access to historical support data, and following an iterative approach are the best ways to evaluate support automation platforms and run a successful POC.