LLM Grounding: Innovating Performance and Productivity
Large Language Models (LLMs) are key innovators in the rapidly advancing field of artificial intelligence, enhancing efficiency and productivity across various sectors through generative AI. LLM grounding is a transformative technique that significantly improves these models by incorporating industry-specific knowledge and data. Grounding AI is crucial for improving the accuracy and contextuality of AI models, enabling them to produce more precise and contextually relevant outputs.
Below is what you will know after this article
Introduction to LLM Grounding
Defining LLM Grounding
Importance of LLM Grounding
Mechanisms of LLM Grounding
Challenges in LLM Grounding
Integrating RAG with LLM Grounding
Using Entity-Based Data Products
Applications and Future Prospects
Conclusion
Introduction to Grounding Large Language Models
Revolutionizing AI with Domain Expertise
Large Language Models (LLMs) are central to many advancements in artificial intelligence, driving efficiency and productivity by understanding and generating human-like text. However, their true potential is unlocked through LLM grounding—a process that embeds them with industry-specific knowledge, making their responses not only accurate but also contextually relevant to specific organizational needs.
Defining LLM Grounding
Enhancing Language Models with Precision
LLM grounding involves enriching large language models with domain-specific information. This enables them to understand and produce responses that are accurate and relevant to specific industries or organizational contexts. By integrating bespoke datasets and knowledge bases, these models are trained to navigate the nuances of specialized terminologies and concepts, significantly enhancing their performance.
During their initial training, LLMs are exposed to extensive datasets from the internet. This process is akin to a broad curriculum, teaching LLMs a wide range of information. However, these models often struggle with industry-specific details and jargon, necessitating the process of grounding to transform them into strategic assets.
Importance of LLM Grounding
Counteracting AI Hallucination
One of the foremost advantages of LLM grounding is its ability to mitigate “AI Hallucination”—where models generate misleading or incorrect responses due to flawed training data or misinterpretation. By using actual web search results, AI models can provide factual responses based on reliable and up-to-date sources. Grounded models have a context-aware foundation, reducing inaccuracies and ensuring reliable, fact-based outputs.
Enhancing Understanding
Grounded LLMs exhibit a superior understanding of complex topics and language nuances unique to specific industries. This improved comprehension allows AI models to interact more effectively with users, guiding them through complex inquiries and clarifying intricate issues. Using relevant technical documentation can further enhance this understanding by providing context and grounding the AI models.
Improving Accuracy and Efficiency
By incorporating industry-specific knowledge, LLM grounding ensures that AI models can provide more accurate and relevant solutions quickly. This precision stems from a deep understanding of the unique challenges within specific sectors, enhancing overall operational efficiency.
Accelerating Problem-Solving
Grounded models, with their enriched knowledge base, can quickly identify and address complex issues, reducing resolution times and streamlining problem-resolution processes across the enterprise.
Mechanisms of LLM Grounding
Transforming AI Models with Domain Expertise
LLM grounding revolutionizes how LLMs understand and interact within specific enterprise contexts by infusing them with domain-specific knowledge. This process involves several meticulously designed stages:
Grounding with Lexical Specificity
This foundational step tailors the LLM to the specific lexical and conceptual framework of an enterprise. Data sources include:
Enterprise-grade ontologies: Structures that encapsulate the enterprise’s lexicon and terms.
Service Desk Tickets: Problem-solving instances and solutions that enrich the model’s practical understanding of common issues.
Conversation Logs and Call Transcripts: Real-world communication samples that enhance the model’s grasp of language patterns.
Vector databases enable semantic search by storing word embeddings as vectors, allowing for more relevant and accurate retrieval of information to improve grounding and responses from the AI models.
Grounding with Unexplored Data
This stage incorporates new and diverse datasets not part of the initial model training, addressing biases and broadening the model’s knowledge base with industry-specific public resources and proprietary content.Using multiple internet search queries can retrieve diverse information, improving the grounding process by providing factual responses and reliable sources.
Grounding with Multi-Content-Type Data
LLM grounding also involves teaching the model to interpret and process various data formats, crucial for tasks like content comprehension, information extraction, and summarization.
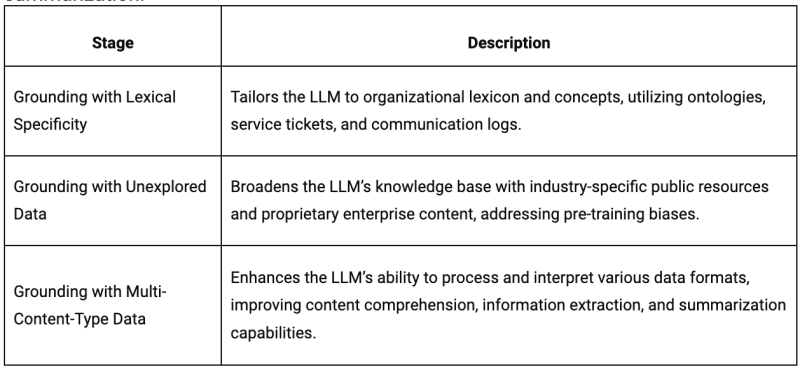
Challenges in LLM Grounding
Overcoming Hurdles to Achieve Precision
Challenges in LLM grounding primarily revolve around the complexity of integrating diverse and specialized data into a cohesive learning framework. Sourcing and curating high-quality, domain-specific data require extensive expertise and resources. Additionally, ensuring data relevance and mitigating biases inherent in training data are critical tasks. Maintaining context, such as using multimodal features like visual input, is essential for effective responses and overcoming grounding challenges.
Integrating RAG with LLM Grounding
Enhancing Real-Time AI Responses
Retrieval-augmented generation (RAG) enhances LLM grounding by dynamically incorporating external data during response generation. By utilizing web search results, RAG can provide factual and reliable responses based on current information found through web searches. This approach enables LLMs to access the most relevant information from a vast database at runtime, ensuring contextually appropriate and up-to-date responses.
Implementing RAG, however, presents challenges, including efficient data retrieval and managing data relevance and accuracy. Despite these hurdles, RAG significantly amplifies LLM performance, especially in scenarios requiring real-time access to expansive knowledge bases.
Using Entity-Based Data Products
Precision Through Structured Knowledge
Grounding LLMs using entity-based data products involves integrating structured data about specific entities (such as people, places, organizations, and concepts) to improve the model's comprehension and output. This method allows LLMs to have a nuanced understanding of entities, their attributes, and their relationships, enabling more precise and informative responses.
The challenge lies in curating and maintaining an extensive, up-to-date entity database that accurately reflects the complexity of real-world interactions. Integrating this structured knowledge into the inherently unstructured learning process of LLMs requires innovative approaches to model training and data integration.
Applications and Future Prospects
Realizing the Potential of Grounded AI
LLM grounding is not just a theoretical concept but a practical tool that significantly enhances AI applications in various fields. Grounded LLMs find applications in IT support, HR processes, procurement, and many other areas, providing precise, contextually aware responses that streamline operations and improve decision-making.Real world examples include using grounded AI in healthcare to assist in diagnosing diseases, in finance to detect fraudulent activities, and in customer service to provide more accurate and helpful responses.
A Vision for the Future
As enterprises continue to integrate AI into their operations, the importance of grounding LLMs will only grow. Future developments may include more sophisticated grounding techniques, greater integration with real-time data sources, and enhanced capabilities for understanding and generating multimodal content.
Conclusion
Embracing Grounded AI for Enterprise Excellence
LLM grounding is a pivotal innovation, steering enterprises toward leveraging AI’s potential for remarkable efficiencies. This strategy enhances base language models with deep, industry-specific knowledge, making it an indispensable tool in the dynamic field of AI.
By enriching comprehension, delivering precise solutions, rectifying AI misconceptions, and expediting problem-solving, LLM's grounding significantly contributes to various facets of enterprise operations. It empowers organizations to transcend the inherent limitations of base models, equipping them with AI capabilities that deeply understand and interact within their unique business contexts.
As we navigate the complex terrain of AI integration in business, adopting LLM grounding emerges as essential, heralding a future where AI and human expertise converge to drive unprecedented advancement. Book a free demo and see for yourself how IrisAgent’s LLMs are revolutionizing customer support.